1 Bayesian Belief Network Bbn Solved Numerical Example Burglar Alarm System By Mahesh Huddar
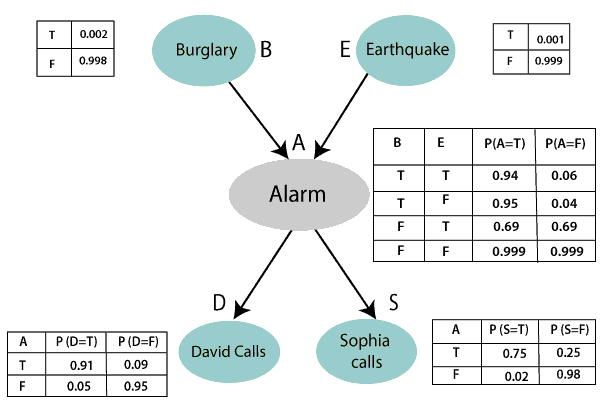
Bayesian Belief Network In Artificial Intelligence Javatpoint 1. bayesian belief network (bbn) solved numerical example burglar alarm system by mahesh huddarexample 2: youtu.be iz7kl2gcmlkyou have a new burgl. 2. bayesian belief network | bbn | solved numerical example burglar alarm system by mahesh huddaryou have a new burglar alarm installed at home.it is fairly.
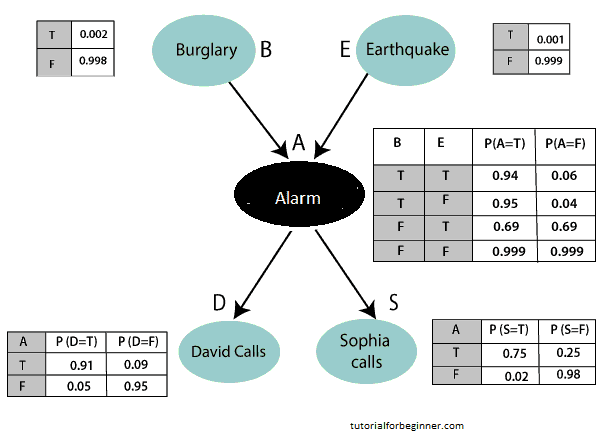
Bayesian Belief Network In Artificial Intelligence 3. bayesian belief network bbn solved numerical example battery gauge fuel and start car by mahesh huddar*****the following concep. Modeling uncertainty with probabilities. knowledge based system era (70s – early 80’s) extensional non probabilistic models. solve the space, time and acquisition bottlenecks in probability based models. froze the development and advancement of kb systems and contributed to the slow down of ai in 80s in general. Bayesian networks. a bn consists of a directed acyclic graph (dag) and. set of conditional probability distributions. in the dag. each node denotes random a variable. each edge from x to y represents that x directly influences y. formally: each variable x is independent of its non descendants given its parents. Basic understanding of bayesian belief networks.
Bayesian Belief Networks Bayesian networks. a bn consists of a directed acyclic graph (dag) and. set of conditional probability distributions. in the dag. each node denotes random a variable. each edge from x to y represents that x directly influences y. formally: each variable x is independent of its non descendants given its parents. Basic understanding of bayesian belief networks. Inference in bayesian networks. computing: p ( j t ) approach 1. blind approach. sum out all un instantiated variables from the full joint, express the joint distribution as a product of conditionals. ( j t ) . p ( b b , e e , a a , j t , m m ) b t , f e t , f a t , f m t , f. 1 cs 2750 machine learning cs 2740 knowledge representation lecture 20 milos hauskrecht [email protected] 5329 sennott square bayesian belief networks: inference cs 2750 machine learning bayesian belief network. burglary earthquake johncalls marycalls alarm p(b) p(e) p(a|b,e) p(j|a) p(m|a) 1. directed acyclic graph • nodes = random variables.
Comments are closed.