All You Need юааto Knowюаб About юааcanadaюабтащюааsюаб юааnewюаб
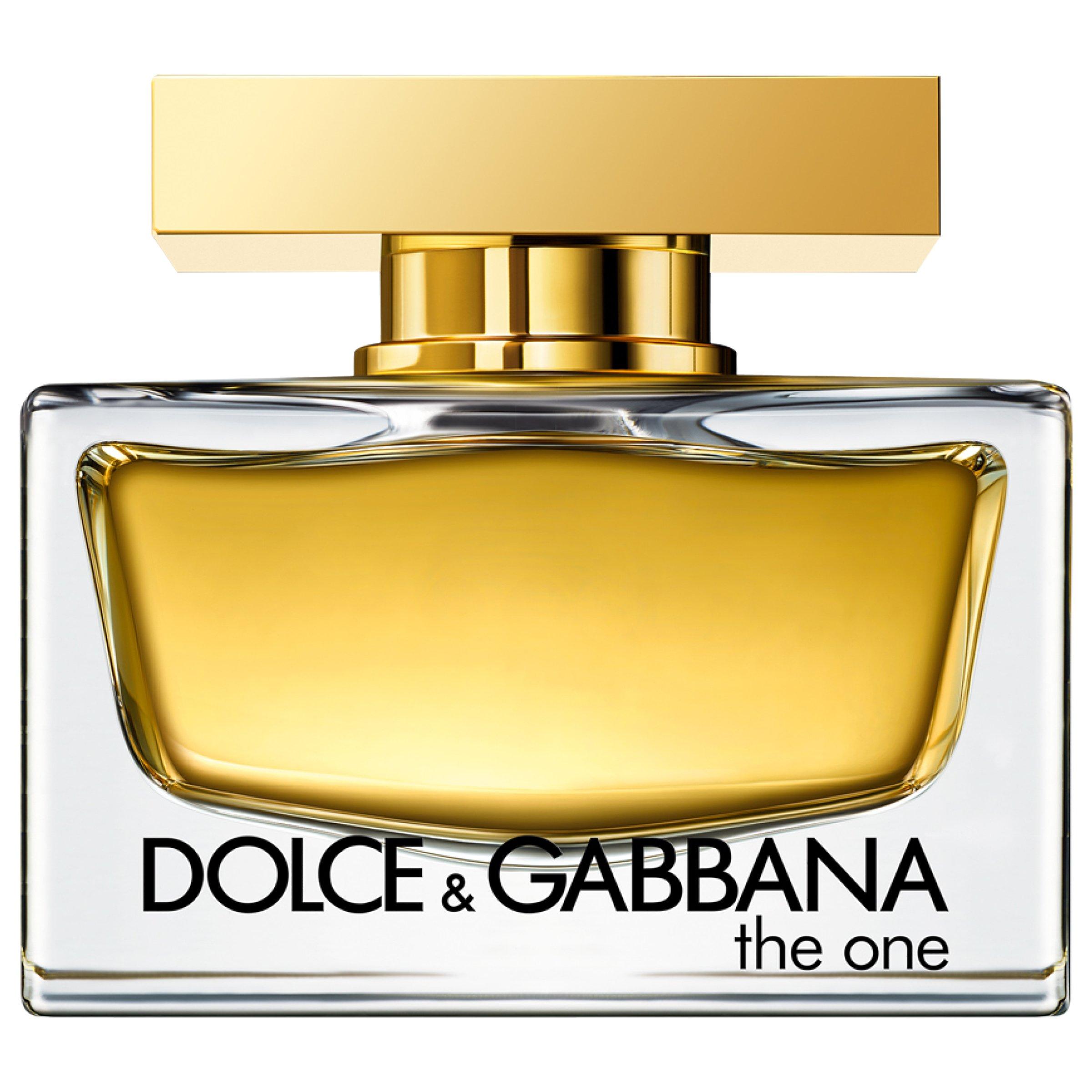
The One Edp 1882400 Dolce Gabbana While single head attention is 0.9 bleu worse than the best setting, quality also drops off with too many heads. 5we used values of 2.8, 3.7, 6.0 and 9.5 tflops for k80, k40, m40 and p100, respectively. table 3: variations on the transformer architecture. unlisted values are identical to those of the base model. An illustration of main components of the transformer model from the paper. " attention is all you need " [1] is a 2017 landmark [2][3] research paper in machine learning authored by eight scientists working at google. the paper introduced a new deep learning architecture known as the transformer, based on the attention mechanism proposed in.

A Painting Of Many Different Animals In The Woods Attention is all you need. ashish vaswani, noam shazeer, niki parmar, jakob uszkoreit, llion jones, aidan n. gomez, lukasz kaiser, illia polosukhin. view a pdf of the paper titled attention is all you need, by ashish vaswani and 7 other authors. the dominant sequence transduction models are based on complex recurrent or convolutional neural. Attention is all you need authors : ashish vaswani , noam shazeer , niki parmar , jakob uszkoreit , 4 , llion jones , aidan n. gomez , Łukasz kaiser , illia polosukhin (less) authors info & claims. Description. default. custom. #2 best model for multimodal machine translation on multi30k (blue (de en) metric) image. default. custom. The best performing models also connect the encoder and decoder through an attention mechanism. we propose a new simple network architecture, the transformer, based solely on attention mechanisms, dispensing with recurrence and convolutions entirely. experiments on two machine translation tasks show these models to be superior in quality while.

трамп надеется выиграть и суд и предстоящие выборы президента Youtube Description. default. custom. #2 best model for multimodal machine translation on multi30k (blue (de en) metric) image. default. custom. The best performing models also connect the encoder and decoder through an attention mechanism. we propose a new simple network architecture, the transformer, based solely on attention mechanisms, dispensing with recurrence and convolutions entirely. experiments on two machine translation tasks show these models to be superior in quality while. Itself. to facilitate these residual connections, all sub layers in the model, as well as the embedding layers, produce outputs of dimension d model = 512. decoder: the decoder is also composed of a stack of n= 6 identical layers. in addition to the two sub layers in each encoder layer, the decoder inserts a third sub layer, which performs. The transformer uses multi head attention in three different ways: 1) in “encoder decoder attention” layers, the queries come from the previous decoder layer, and the memory keys and values come from the output of the encoder. this allows every position in the decoder to attend over all positions in the input sequence.

рќрћр рћ 2015 р р рјсѓрєрё с рѕрї All I Need сѓ Louboutin рґрёр р р рѕ рџрѕсђсљс р р рјр Itself. to facilitate these residual connections, all sub layers in the model, as well as the embedding layers, produce outputs of dimension d model = 512. decoder: the decoder is also composed of a stack of n= 6 identical layers. in addition to the two sub layers in each encoder layer, the decoder inserts a third sub layer, which performs. The transformer uses multi head attention in three different ways: 1) in “encoder decoder attention” layers, the queries come from the previous decoder layer, and the memory keys and values come from the output of the encoder. this allows every position in the decoder to attend over all positions in the input sequence.
Comments are closed.