Beginner S Guide To Quantum Machine Learning Paperspace Blog
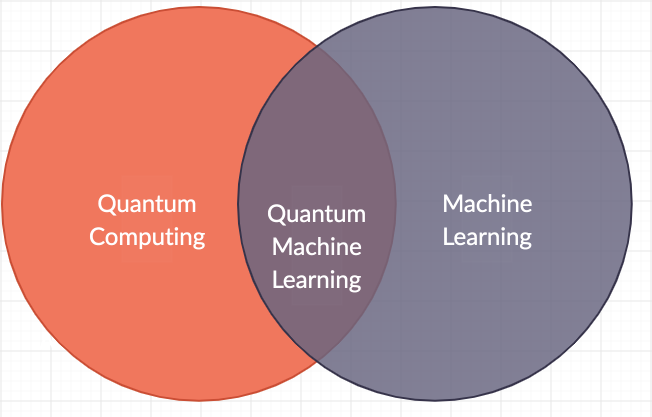
Beginner S Guide To Quantum Machine Learning Paperspace Blog 1) quantum machine learning to solve linear algebraic problems. a wide variety of data analysis and machine learning problems are solved by performing matrix operation on vectors in a high dimensional vector space. in quantum computing, the quantum state of the qubits is a vector in a 2ª dimensional complex vector space. Beginner's guide to quantum machine learning. stay updated with paperspace blog by signing up for our newsletter. your email address join now.
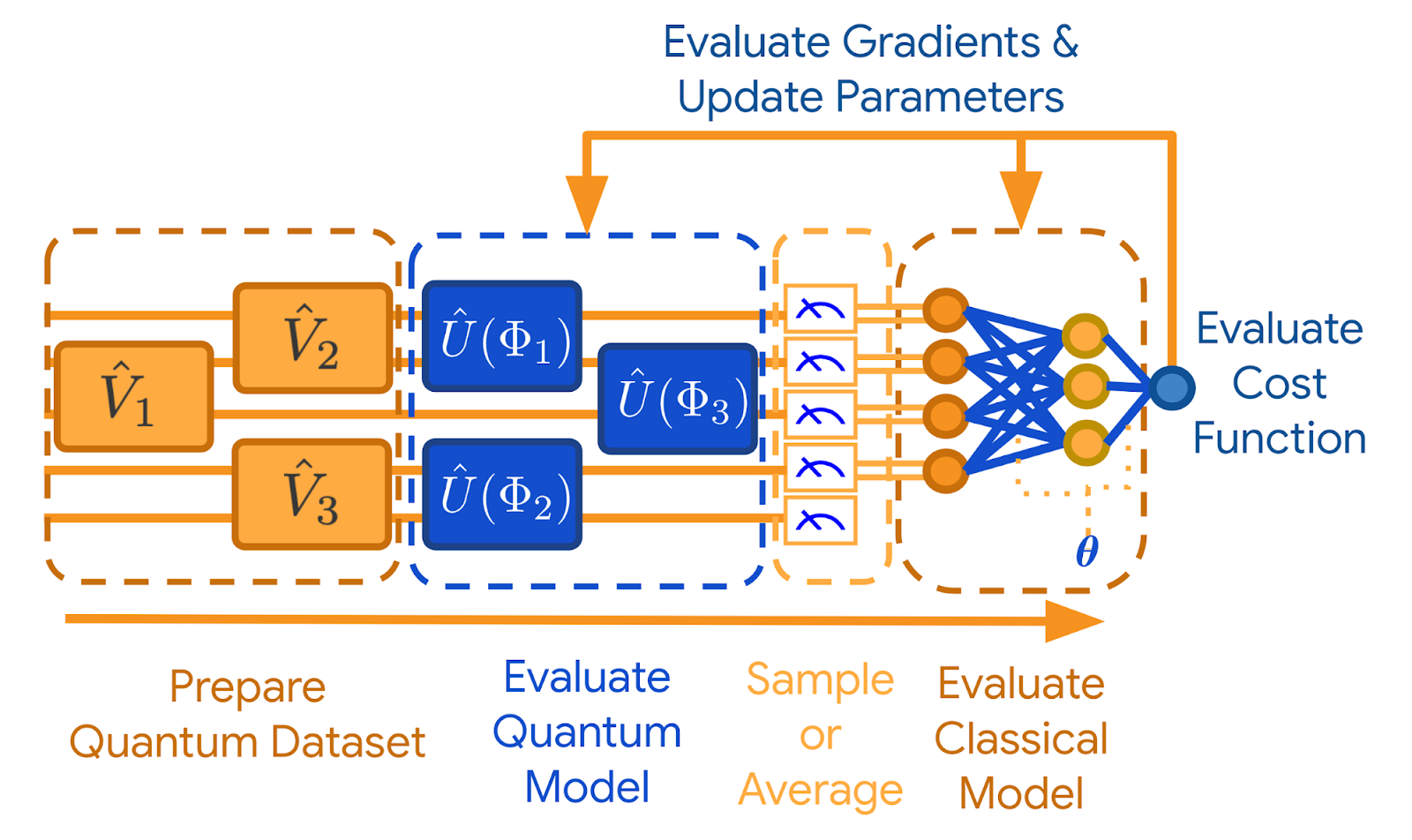
Beginner S Guide To Quantum Machine Learning Paperspace Blog Quantum machine learning explores the application of quantum computing in the fields of ml and dl. this article introduces beginners to the topic, covering the following concepts: a comparison of classical programming, machine learning, and quantum machine learning paradigms. the fundamental concepts of quantum computing. The nodes in boltzmann machines are simply categorized as visible and hidden nodes. the visible nodes take in the input. the same nodes which take in the input will return back the reconstructed input as the output. this is achieved through bidirectional weights which will propagate backwards and render the output on the visible nodes. Step 1: install the necessary libraries and dependencies. for this tutorial, we will be using the pennylane library for quantum machine learning, as well as numpy for numerical computing and. Today, in collaboration with the university of waterloo, x, and volkswagen, we announce the release of tensorflow quantum (tfq), an open source library for the rapid prototyping of quantum ml models. tfq provides the tools necessary for bringing the quantum computing and machine learning research communities together to control and model.
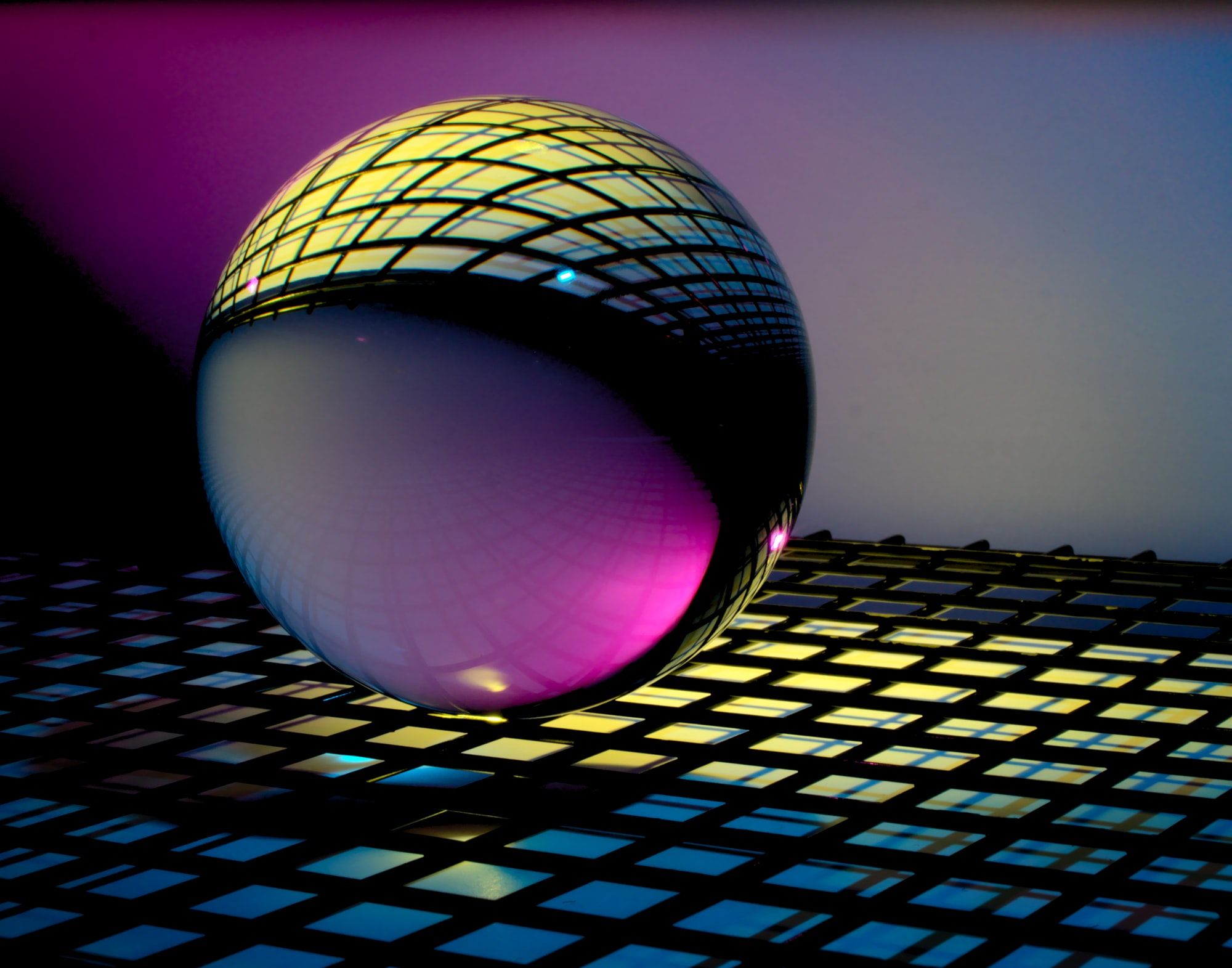
Beginner S Guide To Quantum Machine Learning Paperspace Blog Step 1: install the necessary libraries and dependencies. for this tutorial, we will be using the pennylane library for quantum machine learning, as well as numpy for numerical computing and. Today, in collaboration with the university of waterloo, x, and volkswagen, we announce the release of tensorflow quantum (tfq), an open source library for the rapid prototyping of quantum ml models. tfq provides the tools necessary for bringing the quantum computing and machine learning research communities together to control and model. Also called quantum enhanced machine learning, quantum machine learning leverages the information processing power of quantum technologies to enhance and speed up the work performed by a machine learning model. while classical computers are constrained by limited storage and processing capacities, quantum enabled ones allow for exponentially. This article explains quantum machine learning for beginners, a promising field that applies quantum computing to machine learning and deep learning. principal component analysis wave function.
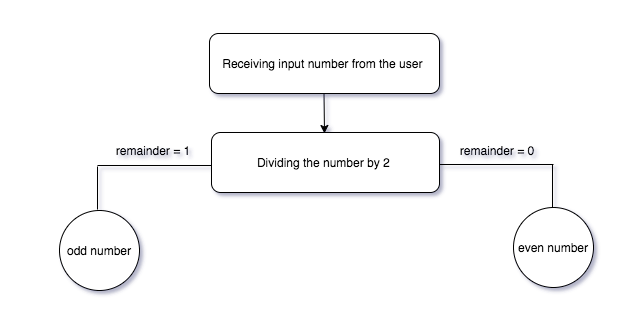
Beginner S Guide To Quantum Machine Learning Paperspace Blog Also called quantum enhanced machine learning, quantum machine learning leverages the information processing power of quantum technologies to enhance and speed up the work performed by a machine learning model. while classical computers are constrained by limited storage and processing capacities, quantum enabled ones allow for exponentially. This article explains quantum machine learning for beginners, a promising field that applies quantum computing to machine learning and deep learning. principal component analysis wave function.
Comments are closed.