Bias And Fairness In Ai Systems With Lead Machine Learning Developer At Altaml Graham Erickson

Bias And Fairness In Ai Systems With Lead Machine Learning Graham erickson, lead machine learning developer at altaml highlights the need to analyze data through the lens of bias and fairness when developing #aisyste. The new artificial intelligence (ai) technology could improve wildfire fighting and save money and resources. interview with graham erickson, the senior lead machine learning developer at.
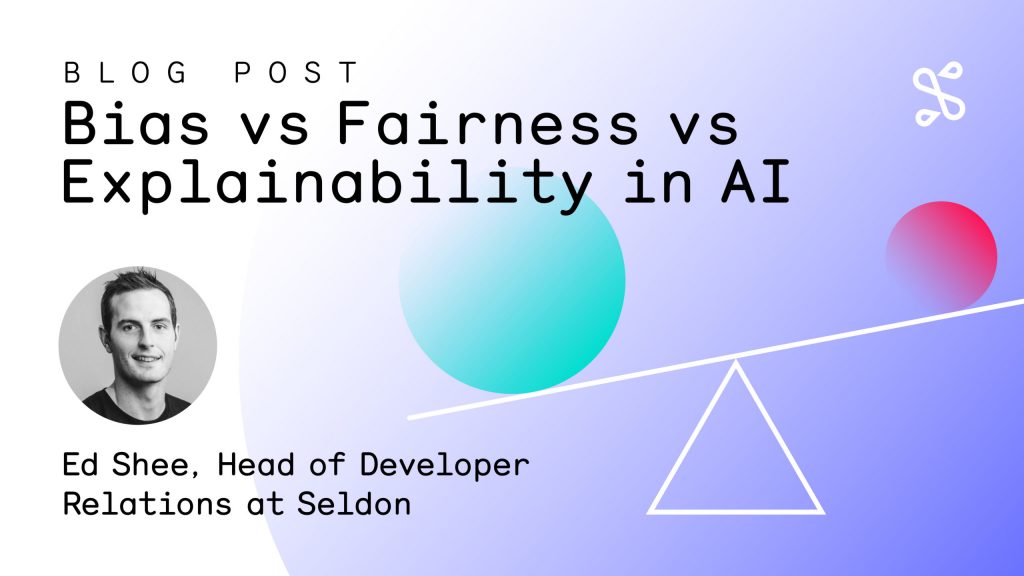
Bias Vs Fairness Vs Explainability In Ai Seldon Mar 24, 2021. 164. 2. when ai makes headlines, all too often it’s because of problems with bias and fairness. some of the most infamous issues have to do with facial recognition, policing, and health care, but across many industries and applications, we’ve seen missteps where machine learning is contributing to creating a society where some. The new artificial intelligence (ai) technology could improve wildfire fighting and save money and resources. to get more information about that, hilary bird was joined by graham erickson. he is. For graham erickson, senior lead machine learning developer at altaml, alberta’s predictive tool highlights the power of ai and public private partnerships. alberta wildfire’s trove of historic data spans decades with details on size, cause, location, start time, duration, weather conditions, staffing, suppression equipment and area burned for every wildfire in the province. This can stimulate the next steps in the use of algorithms and ai. this includes, for example, a trained, enlightened use of algorithmic decision making systems. bias and fairness are only pieces of the puzzle of trustworthy and ethical ai. in addition to bias and fairness, it also includes privacy and accountability.
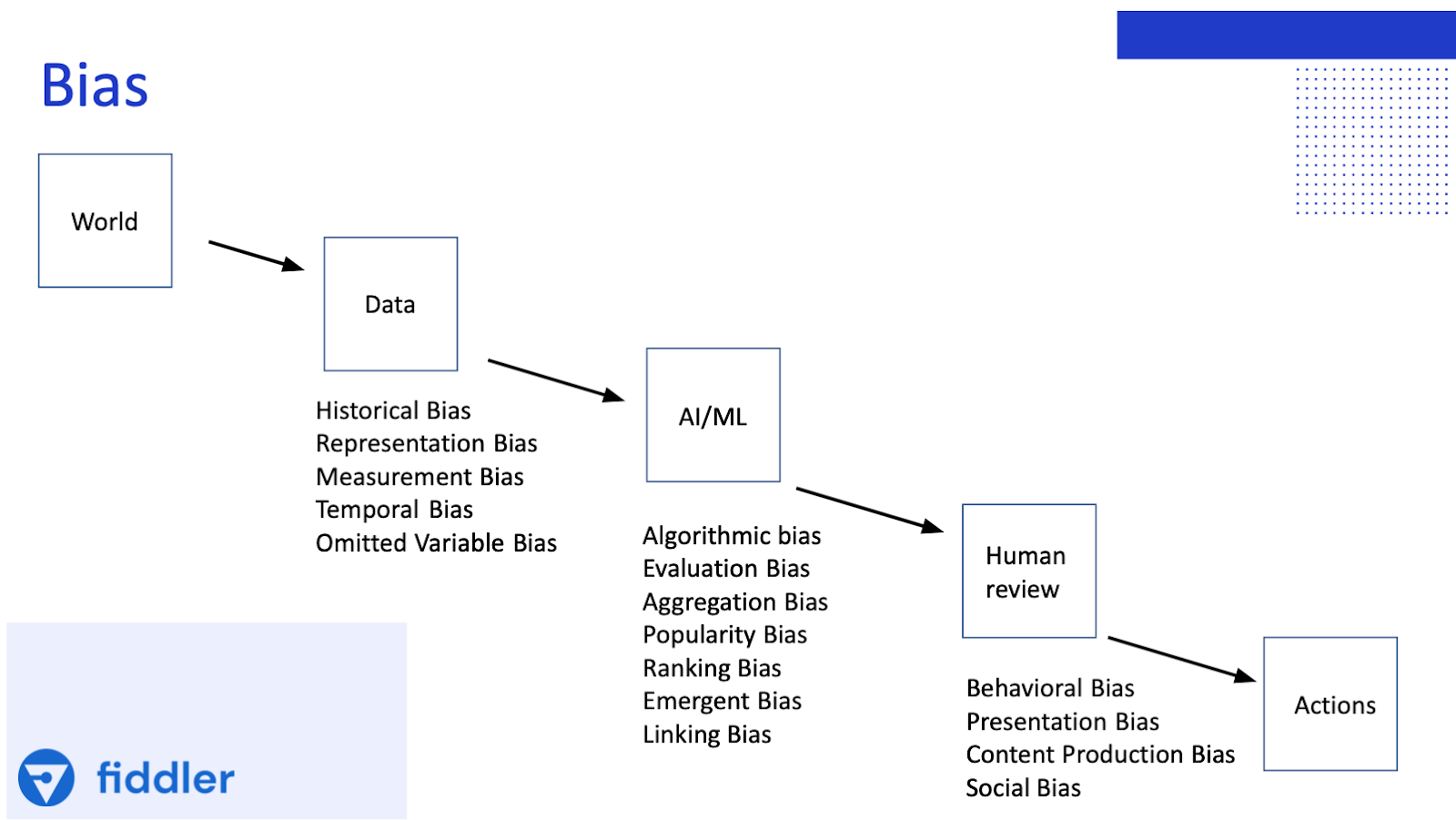
Ai Explained Understanding Bias And Fairness In Ai Systems Fiddler For graham erickson, senior lead machine learning developer at altaml, alberta’s predictive tool highlights the power of ai and public private partnerships. alberta wildfire’s trove of historic data spans decades with details on size, cause, location, start time, duration, weather conditions, staffing, suppression equipment and area burned for every wildfire in the province. This can stimulate the next steps in the use of algorithms and ai. this includes, for example, a trained, enlightened use of algorithmic decision making systems. bias and fairness are only pieces of the puzzle of trustworthy and ethical ai. in addition to bias and fairness, it also includes privacy and accountability. Abstract. sex and gender biases can be entrenched in the life cycle of artificial intelligence (ai) development, from data acquisition to technological design and deployment. ai systems that fail to account for the contribution of sex and gender to health generate suboptimal results and discriminatory outcomes. Graham erickson is a lead machine learning developer at altaml. graham was previously a data scientist for the women and children's health research institute and granify. graham has also been a graduate student and student researcher at the university of alberta and the university of saskatchewan.
Comments are closed.