Disentangled Representation Learning A Latent Traversals Used To

Disentangled Representation Learning A Latent Traversals Used To A latent traversal of a network trained with the β value adjusted during training. a disentangled representation is encoded, while maintaining high quality reconstructions. it does appear to be. Fig. 1 disentangled representation learning. a latent traversals used to visualise the semantic meaning encoded by single disentangled latent units of a trained model.

Disentangled Representation Learning A Latent Traversals Used To Disentangled representation learning aims to improve the explainability of deep learning methods by training a data encoder that identifies semantically meaningful latent variables in the data generation process. nevertheless, there is no consensus regarding a universally accepted definition for the objective of disentangled representation learning. in particular, there is a considerable. Download scientific diagram | disentangled representation learning. a latent traversals used to visualise the semantic meaning encoded by single disentangled latent units of a trained model. in. Formation from the multiple d. mains.disentangled representations. disentanglement representation learning [8, 33] aims at recovering the factors of variations. nderlying a given data distribution. [56] proved that without any form of supervision (whether direct or indirect) on the factors of variation (. Abstract. latent traversal is a popular approach to visualize the disentangled latent representations. given a bunch of variations in a single unit of the latent representation, it is expected that there is a change in a single factor of variation of the data while others are fixed. however, this impressive experimental observation is rarely.

Learning Disentangled Representations вђ Part 1 Simple Dots By David Formation from the multiple d. mains.disentangled representations. disentanglement representation learning [8, 33] aims at recovering the factors of variations. nderlying a given data distribution. [56] proved that without any form of supervision (whether direct or indirect) on the factors of variation (. Abstract. latent traversal is a popular approach to visualize the disentangled latent representations. given a bunch of variations in a single unit of the latent representation, it is expected that there is a change in a single factor of variation of the data while others are fixed. however, this impressive experimental observation is rarely. Learning disentangled representations is a fundamental goal in many facial analytics methods. for example, in the multi view face recognition problem [ 75 ], we can explicitly split the latent features into facial appearance features (who is this person) and viewpoint features (which is the angle the facial image is taken). Learning low dimensional representations of high dimensional data is a fundamental challenge in unsupervised deep learning [5, 43], where the emphasis is put on learning representations that allow for efficient adaptation across a wide range of tasks [5, 28, 43]. disentanglement [5, 27, 28, 43, 60] has shown significant promise in.
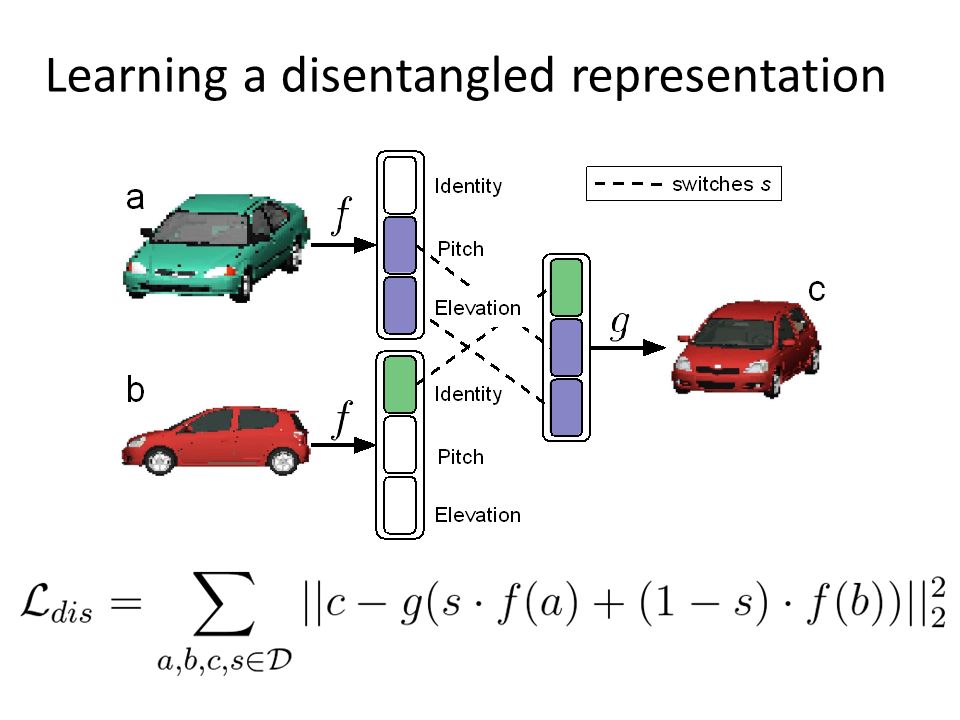
Disentangled Representation Learning Definition Deepai Learning disentangled representations is a fundamental goal in many facial analytics methods. for example, in the multi view face recognition problem [ 75 ], we can explicitly split the latent features into facial appearance features (who is this person) and viewpoint features (which is the angle the facial image is taken). Learning low dimensional representations of high dimensional data is a fundamental challenge in unsupervised deep learning [5, 43], where the emphasis is put on learning representations that allow for efficient adaptation across a wide range of tasks [5, 28, 43]. disentanglement [5, 27, 28, 43, 60] has shown significant promise in.

Network For Disentangled Representation Learning Given In 20
Comments are closed.