Figure 14 From A Novel Handwritten Digit Classification System Based On
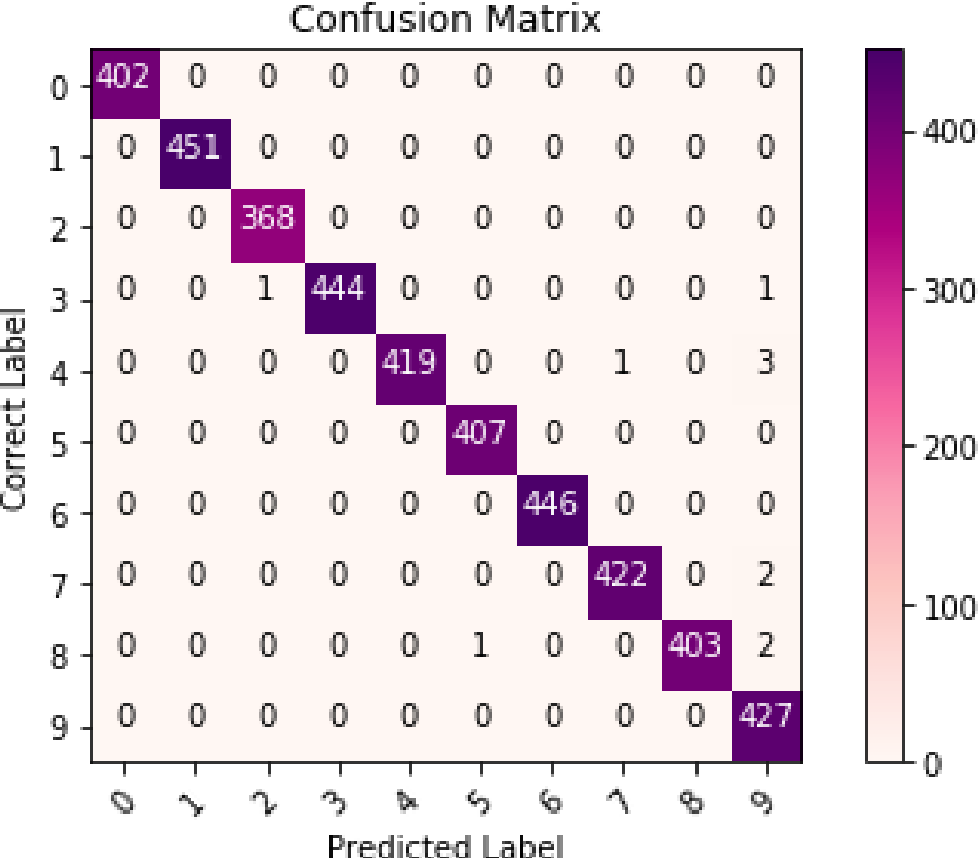
Figure 13 From A Novel Handwritten Digit Classification System о An enormous number of cnn classification algorithms have been proposed in the literature. nevertheless, in these algorithms, appropriate filter size selection, data preparation, limitations in datasets, and noise have not been taken into consideration. as a consequence, most of the algorithms have failed to make a noticeable improvement in classification accuracy. to address the shortcomings. Fourthly, to simulate the real world natural influences that can affect image quality, we propose to add an additive white gaussian noise with σ = 0.5 to the mnist dataset. as a result, our cnn algorithm achieves state of the art results in handwritten digit recognition, with a recognition accuracy of 99.98%, and 99.40% with 50% noise.
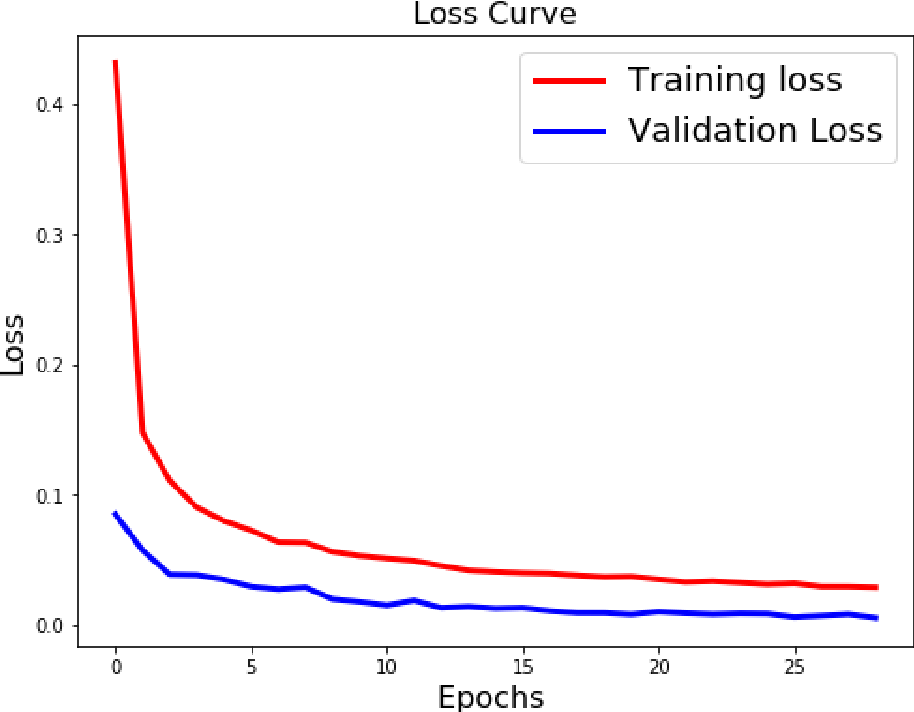
Figure 12 From A Novel Handwritten Digit Classification System о As a result, our cnn algorithm achieves state of the art results in handwritten digit recognition, with a recognition accuracy of 99.98%, and 99.40% with 50% noise. density plot of features in. The size of the effective receptive field (erf) is calculated and the cnn algorithm achieves state of the art results in handwritten digit recognition, with a recognition accuracy of 99.98%, and 99.40% with 50% noise. an enormous number of cnn classification algorithms have been proposed in the literature. nevertheless, in these algorithms, appropriate filter size selection, data preparation. The mnist handwritten digit classification problem is a standard dataset used in computer vision and deep learning. although the dataset is effectively solved, it can be used as the basis for learning and practicing how to develop, evaluate, and use convolutional deep learning neural networks for image classification from scratch. Er engineering, university of victoria (departmental member)abstractin this report, we describe several neur. l network architectures for the classification of handwritten digits. in particular, our attention is focused on the clas. of convolutional neural networks (cnns) for performance superiority. by using matlab deep learning toolbox, we.
Comments are closed.