Harness Your Documents For Genai Build A Rag Pipeline For Data
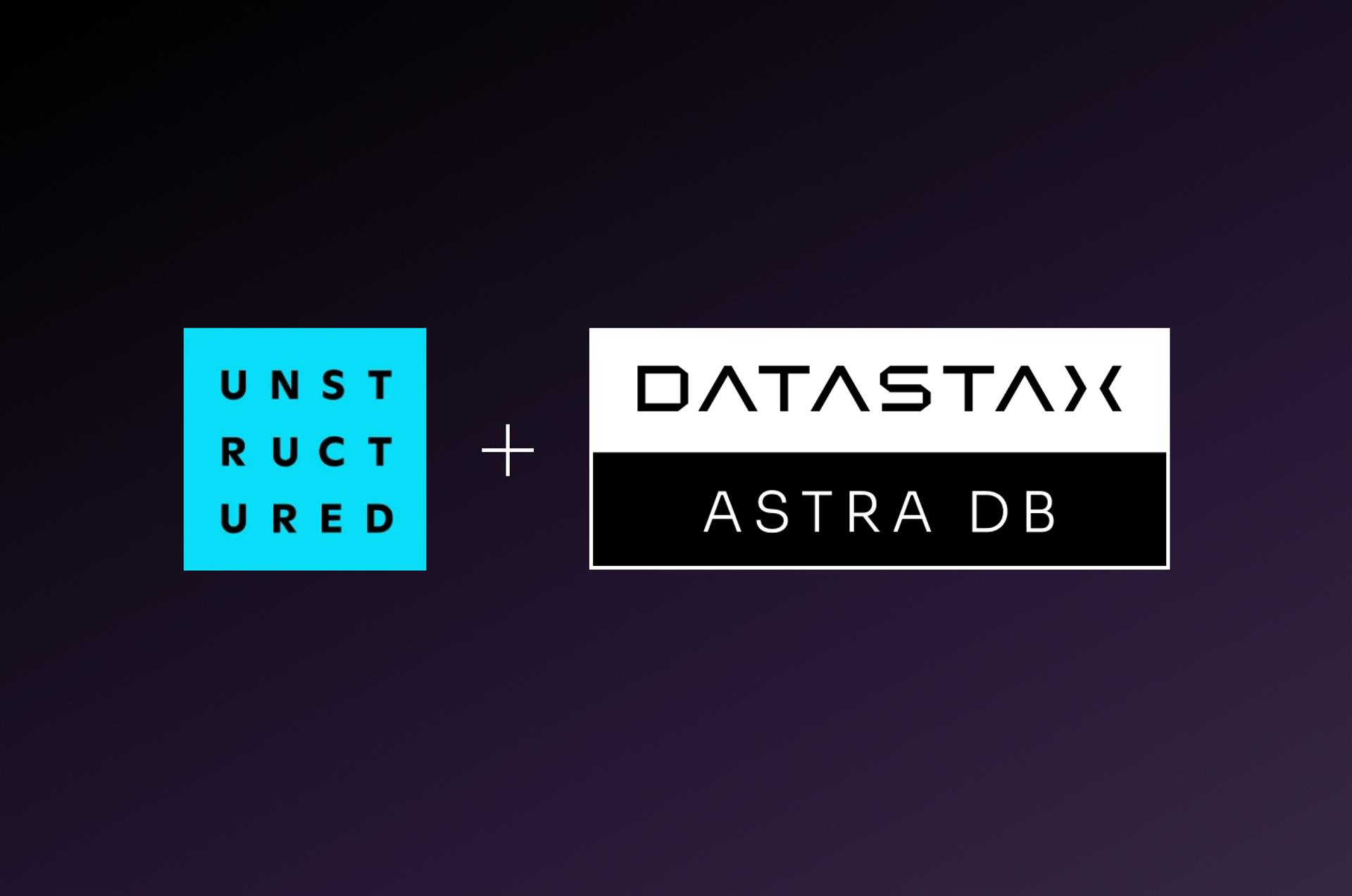
Harness Your Documents For Genai Build A Rag Pipeline Data We’ll build a simple but elegant rag pipeline powered by an integration with astra db that takes a variety of data formats and some simple python code to build an llm based query engine that retrieves parsed data to provide insights to users. to do this we’ll use an astra db integration to unstructured.io as a vector store destination. This delivers: ⚡ lightning fast data ingestion and conversion of documents and data sets into vector data 🔎 embeddings that can be quickly written to astra db for highly relevant genai.
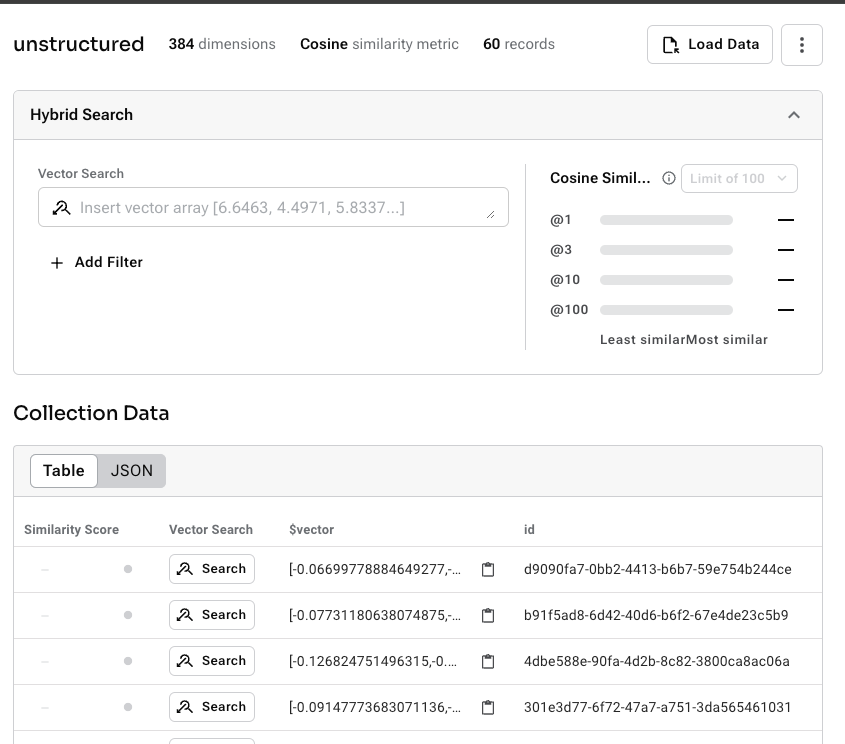
Harness Your Documents For Genai Build A Rag Pipeline Data Introduction. retrieval augmented generation (or rag) pipelines are increasingly becoming the common way to implement question answering and chatbot applications using large language models (llms) with your own data. some of your data may reside on s3 or google drive as files in pdf format or msoffice documents, but in many cases your data is. Apac genai roadshow is coming to bengaluru this june! 🤗 explore real time data for next gen customer experiences with generative ai & rag. learn how ragstack simplifies working with llms. But ragas offers a lot more. different tools and techniques to enable continues learning of your rag application. a core concept that is is worth mentioning, is “component wise evaluation”. ragas offers predefined metrics to evaluate each component of the rag pipeline in isolation, e.g. [ragas, 2024]: generation:. Kendra can connect to multiple data sources and build a unified search index. this allows the rag system to leverage a diverse corpus of documents for passage retrieval. you can use the kendra console to connect to your sources of data and schedule the gathering of the data. alternatively, you can use kendra’s api to do so.
Comments are closed.