Heart Disease Prediction Using Logistic Regression Example Of

Heart Disease Prediction In Tableau Logistic Regression In Tableau Logistic regression is one of the basic and popular algorithms to solve a binary classification problems. for each input, logistic regression outputs a probability that this input belongs to the 2 classes. set a probability threshold boundary and that determines which class the input belongs to. The logistic regression achieved accuracy of 85.71 [8]. thanuja nishadi a s et al., proposed logistic regression model for classification of heart disease on framingham dataset with 4238 rrecords. the logistic regression achieved the accuracy of 86.66% [9]. montu saw et al., proposed logistic regression model to classify the cardiac disease.

Datamaind Interactive Tableau Viz Heart Disease Prediction Based On Logistic regression to predict heart disease using r. we can observe that around 124 patients in the age of 50 60 had no heart diseases while around 100 patients in the age of 50 60 were suffering from heart diseases. this shows there is higher count of people without heart disease in ages of 50 60 in our dataset. Accessible medical data on heart disease. there are. 208 entries in this dataset, and each contains eight. details on the patient, including their age, type of. chest pain, blood sugar lev el, blo. Logistic regression is a statistical and machine learning technique classifying records of a dataset based on the values of the input fields. it predicts a dependent variable based on one or more sets of independent variables to predict outcomes. it can be used both for binary classification and multi class classification. Heart disease cases nearly doubled over the period, from 271 million in 1990 to 523 million in 2019, and the number of heart disease deaths rose from 12.1 million to 18.6 million.

Heart Disease Prediction Using Logistic Regression Example Of Logistic regression is a statistical and machine learning technique classifying records of a dataset based on the values of the input fields. it predicts a dependent variable based on one or more sets of independent variables to predict outcomes. it can be used both for binary classification and multi class classification. Heart disease cases nearly doubled over the period, from 271 million in 1990 to 523 million in 2019, and the number of heart disease deaths rose from 12.1 million to 18.6 million. 2.3 model for predicting risk of a patient having heart disease. logistic regression is a popular statistical method used for analyzing data and building predictive models. this model is used to estimate the probability of a binary outcome (i.e., a binary response variable) based on one or more predictor variables. For example, a patient with an lad value of 38, absence of shortness, <7 days in hospital, mild dependence on adl and no nitrates was predicted to have a risk of 90 day readmission of 29.2% (the best cut off of prediction probabilities in the xgboost model was 46.8%), indicating that the patient was at low risk of 90 day readmission (figure 4d).
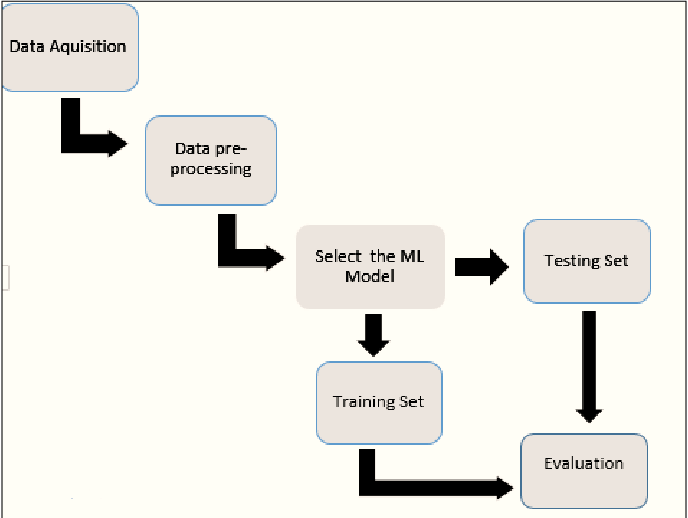
Figure 2 From Predicting Heart Diseases In Logistic Regression Of 2.3 model for predicting risk of a patient having heart disease. logistic regression is a popular statistical method used for analyzing data and building predictive models. this model is used to estimate the probability of a binary outcome (i.e., a binary response variable) based on one or more predictor variables. For example, a patient with an lad value of 38, absence of shortness, <7 days in hospital, mild dependence on adl and no nitrates was predicted to have a risk of 90 day readmission of 29.2% (the best cut off of prediction probabilities in the xgboost model was 46.8%), indicating that the patient was at low risk of 90 day readmission (figure 4d).
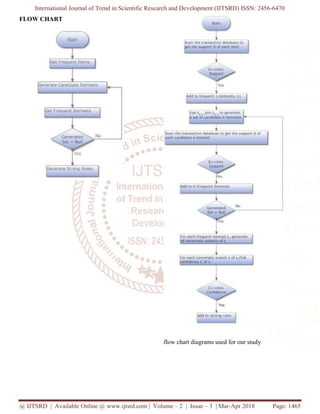
A Heart Disease Prediction Model Using Logistic Regression Pdf
Comments are closed.