How To Go About Interpreting Regression Cofficients
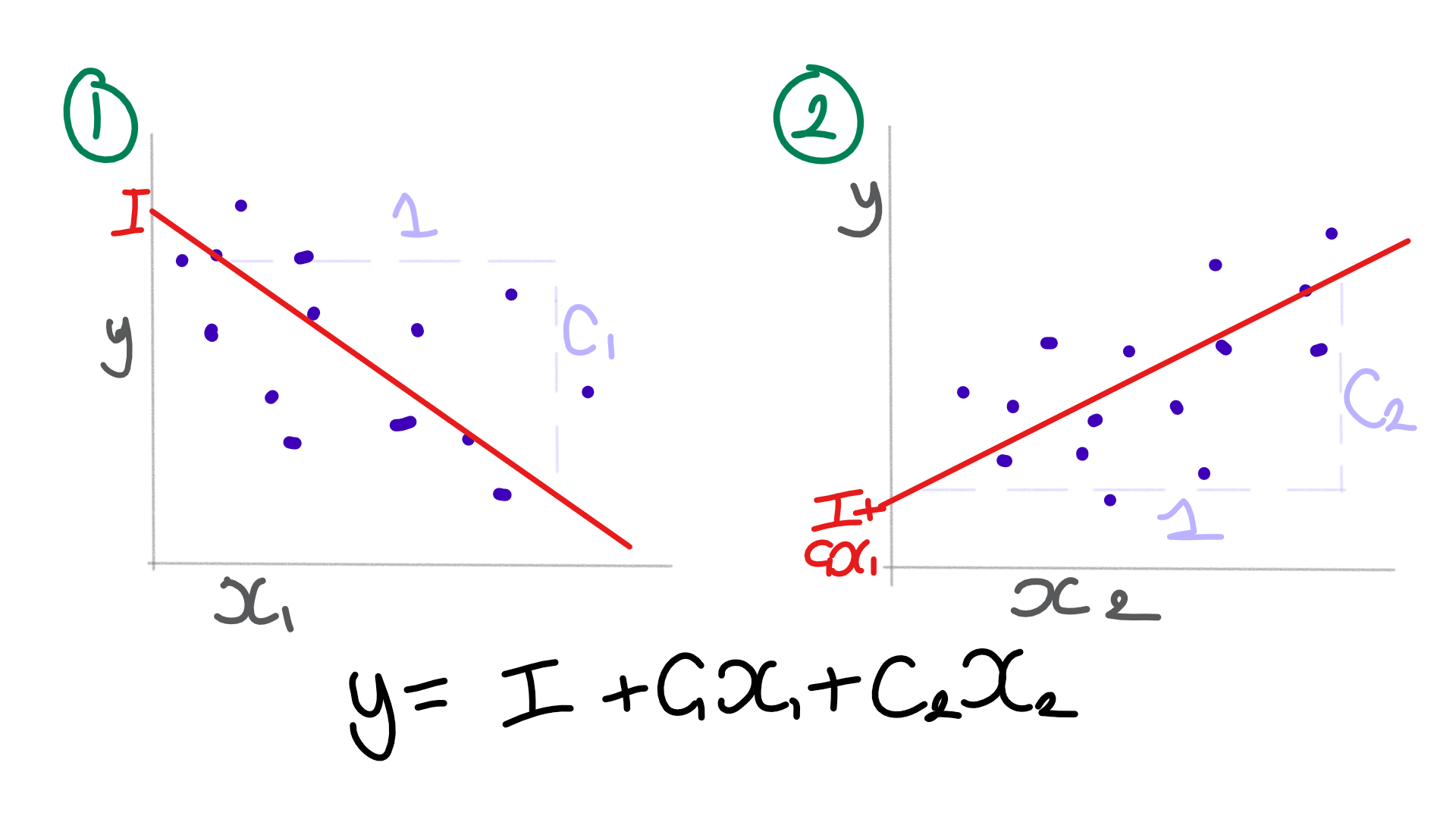
How To Go About Interpreting Regression Cofficients Coefficients are what a line of best fit model produces. a line of best fit (aka regression) model usually consist of an intercept (where the line starts) and the gradients (or slope) for the line for one or more variables. when we perform a linear regression in r, it’ll output the model and the coefficients. each value represents the. Interpreting the intercept. the intercept term in a regression table tells us the average expected value for the response variable when all of the predictor variables are equal to zero. in this example, the regression coefficient for the intercept is equal to 48.56. this means that for a student who studied for zero hours (hours studied = 0.

Interpreting Coefficients In Regressions Youtube Coefficients are what a line of best fit model produces. a line of best fit (aka regression) model usually consist of an intercept (where the line starts) and the gradients (or slope) for the line for one or more variables. when we perform a linear regression in r, it’ll output the model and the coefficients. call:. The height coefficient in the regression equation is 106.5. this coefficient represents the mean increase of weight in kilograms for every additional one meter in height. if your height increases by 1 meter, the average weight increases by 106.5 kilograms. the regression line on the graph visually displays the same information. However, this integration introduces new complexities into the model. the interaction effects between features like “grlivarea” and “neighborhood” can significantly alter the coefficients. for instance, the coefficient for “grlivarea” decreased from 110.52 in the single feature model to 78.93 in the combined model. In the case of a level regression (no log transformation), with the regression coefficients corresponding to a partial derivative (∂ y ∂ x ), a change of one unit in x implies a change of β₁ unit in y (with y the dependent variable, x the independent variable, and β₁ the regression coefficient associated with x).
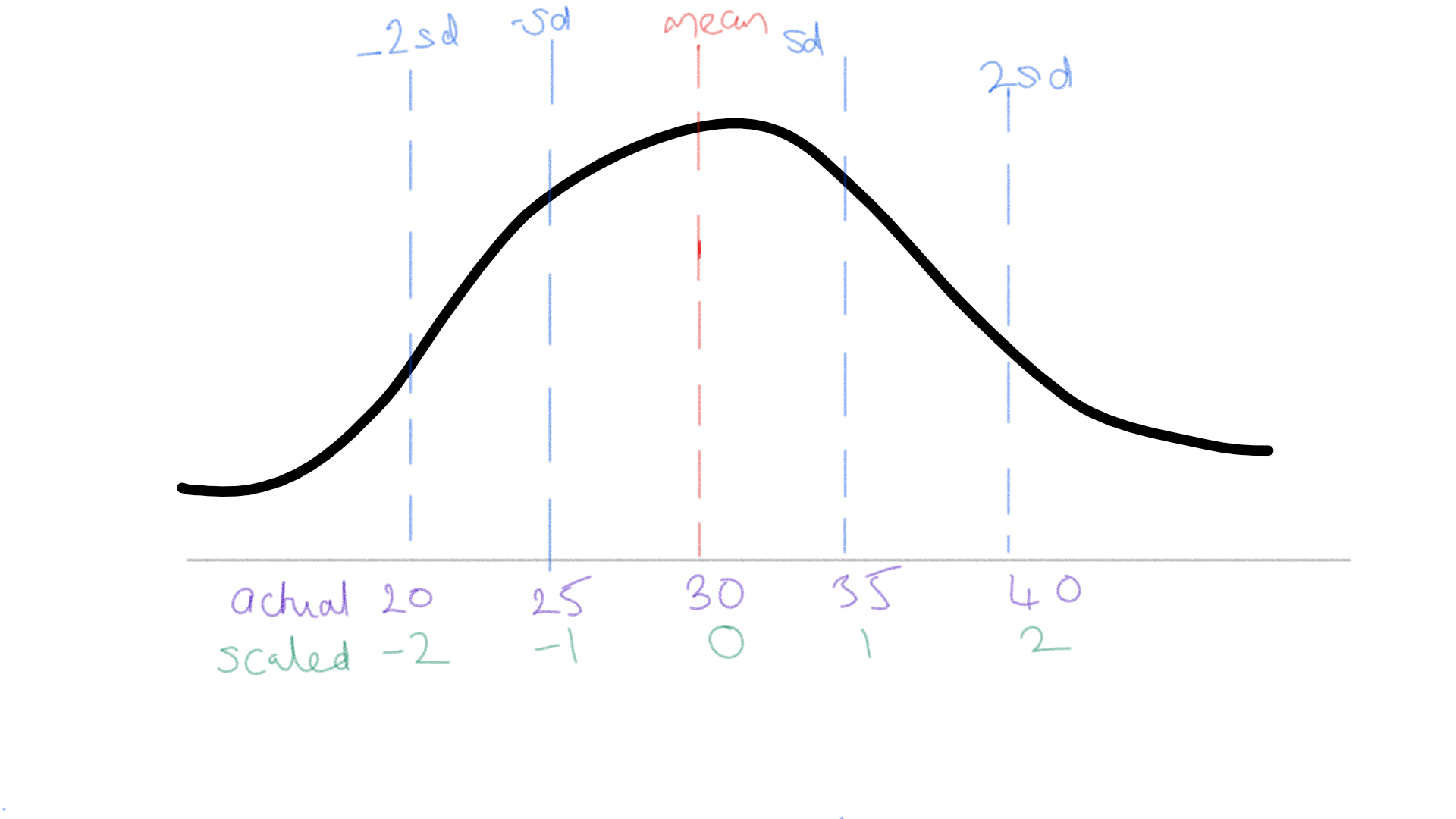
How To Go About Interpreting Regression Cofficients However, this integration introduces new complexities into the model. the interaction effects between features like “grlivarea” and “neighborhood” can significantly alter the coefficients. for instance, the coefficient for “grlivarea” decreased from 110.52 in the single feature model to 78.93 in the combined model. In the case of a level regression (no log transformation), with the regression coefficients corresponding to a partial derivative (∂ y ∂ x ), a change of one unit in x implies a change of β₁ unit in y (with y the dependent variable, x the independent variable, and β₁ the regression coefficient associated with x). Following my post about logistic regressions, ryan got in touch about one bit of building logistic regressions models that i didn’t cover in much detail – interpreting regression coefficients. this post will hopefully help ryan (and others) out. this was so helpful. thank you! i'd love to see more about interpreting the glm coefficients. A linear regression model with two predictor variables results in the following equation: y i = b 0 b 1 *x 1i b 2 *x 2i e i. the variables in the model are: e, the residual error, which is an unmeasured variable. the parameters in the model are: b 2, the second regression coefficient.
Comments are closed.