Interpreting Regression Coefficients In Linear Regression
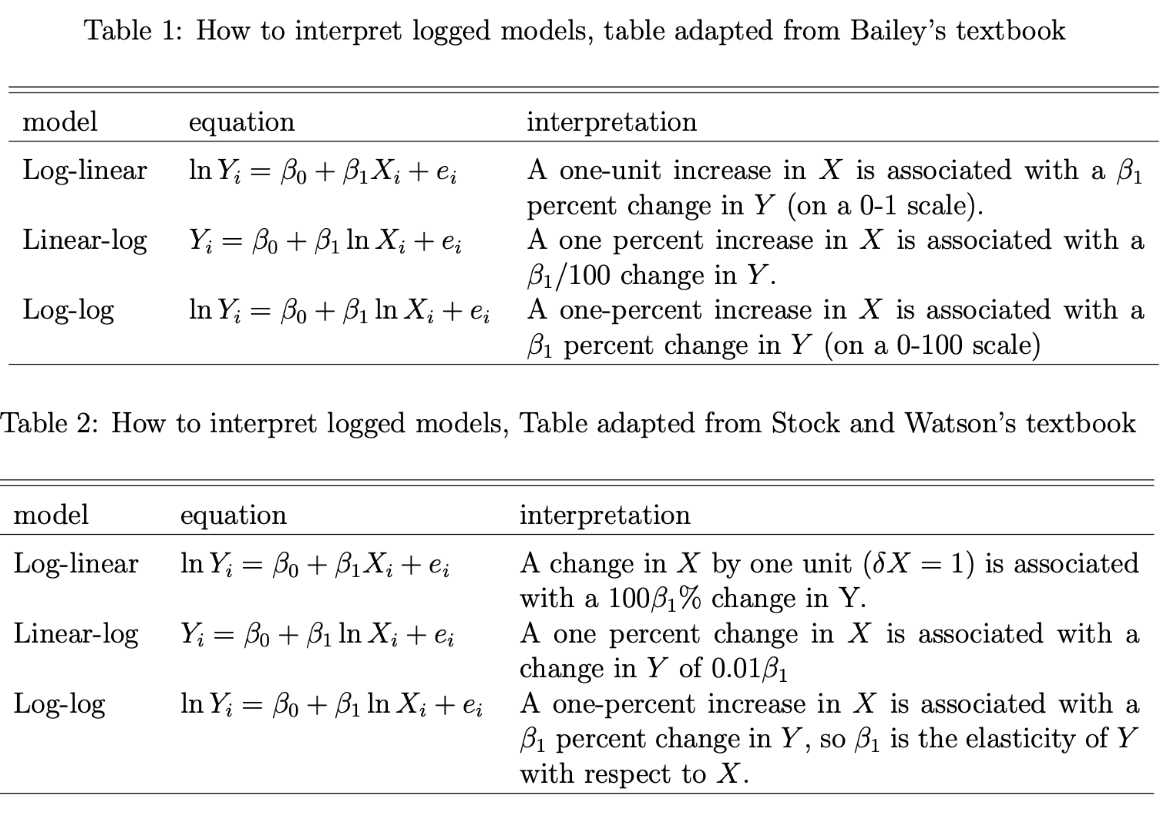
Interpretation Of Regression Coefficients With Examples Interpreting the intercept. the intercept term in a regression table tells us the average expected value for the response variable when all of the predictor variables are equal to zero. in this example, the regression coefficient for the intercept is equal to 48.56. this means that for a student who studied for zero hours (hours studied = 0. The p values for the coefficients indicate whether these relationships are statistically significant. after fitting a regression model, check the residual plots first to be sure that you have unbiased estimates. after that, it’s time to interpret the statistical output. linear regression analysis can produce a lot of results, which i’ll.

Interpretation Of The Multiple Linear Regression Coefficients In Interpreting coefficients in linear models with a single numerical feature. in this section, we focus on a single numerical feature from the ames housing dataset, “grlivarea” (above ground living area in square feet), to understand its direct impact on “saleprice”. we employ k fold cross validation to validate our model’s performance. Looking online at how to interpret linear regression coefficients is similar to looking at how to import a csv file in python, many people can get this information in their heads. after teaching statistics to over 10,000 students for a decade, i still sometimes have to double check the interpretation in some special cases (e.g., a binary result and a log transformed explanatory variable). The example here is a linear regression model. but this works the same way for interpreting coefficients from any regression model without interactions. a linear regression model with two predictor variables results in the following equation: y i = b 0 b 1 *x 1i b 2 *x 2i e i. the variables in the model are: y, the response variable;. The next section shows the degrees of freedom, the sum of squares, mean squares, f statistic, and overall significance of the regression model. here is how to interpret each of the numbers in this section: regression degrees of freedom. this number is equal to: the number of regression coefficients – 1.
Comments are closed.