Lecture 21 Eigenvalues And Eigenvectors
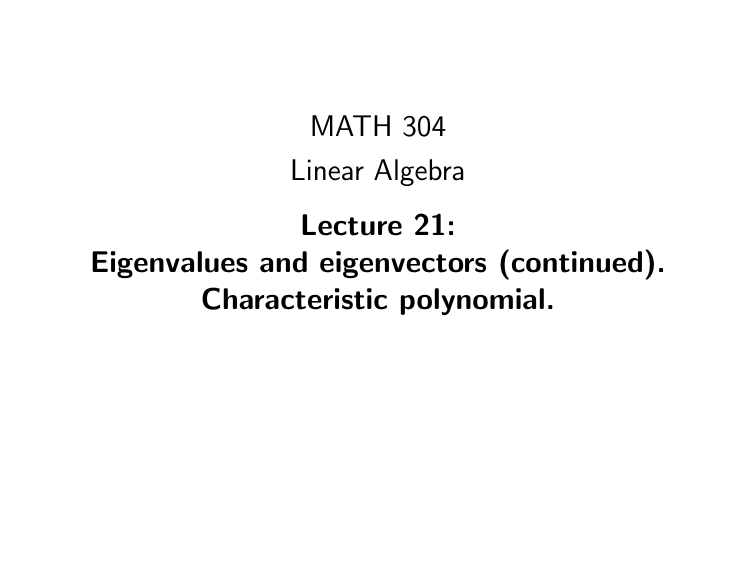
Math 304 Linear Algebra Lecture 21 Eigenvalues And Eigenvectors Lecture 21: eigenvalues and eigenvectors. if the product ax points in the same direction as the vector x, we say that x is an eigenvector of a. eigenvalues and eigenvectors describe what happens when a matrix is multiplied by a vector. in this session we learn how to find the eigenvalues and eigenvectors of a matrix. In general, the eigenvalues of a two by two matrix are the solutions to: λ2 − trace(a) · λ det a = 0. just as the trace is the sum of the eigenvalues of a matrix, the product of the eigenvalues of any matrix equals its determinant. 3 1 for a = , the eigenvalues are λ1 = 4 and λ2 = 2. we find the.
Lecture 4 Eigenvalues And Eigenvectors Pdf Mit 18.06 linear algebra, spring 2005instructor: gilbert strangview the complete course: ocw.mit.edu 18 06s05 playlist:. Eigenvalues and eigenvectors diagonalization and powers of a lecture 21: eigenvalues and eigenvectors. viewing videos requires an internet connection. The eigenvectors corresponding to λ1 = 3 are the vectors. 1) α(2, = v1 α ∈ r. this is a family of vectors with having same direction, that is, they form a linearly dependent set. simlarly, for λ2 = −2, we solve 4 2 x1. (a − (−2) i)x = 2 1 x2. = 0. 0 again the system reduces to a single equation. Function f from example 8.1.2 has no eigenvectors and no eigenvalues, whereas the one from example 8.1.3 has them. as we shall see once we learn how to actually compute eigenvalues and eigenvectors (this will involve finding roots of polynomials), the essential difference is thatc is an algebraically closed field, whereasr is not.

Lecture 11 Eigenvalues And Eigenvectors Part 3 Example Youtube The eigenvectors corresponding to λ1 = 3 are the vectors. 1) α(2, = v1 α ∈ r. this is a family of vectors with having same direction, that is, they form a linearly dependent set. simlarly, for λ2 = −2, we solve 4 2 x1. (a − (−2) i)x = 2 1 x2. = 0. 0 again the system reduces to a single equation. Function f from example 8.1.2 has no eigenvectors and no eigenvalues, whereas the one from example 8.1.3 has them. as we shall see once we learn how to actually compute eigenvalues and eigenvectors (this will involve finding roots of polynomials), the essential difference is thatc is an algebraically closed field, whereasr is not. Most 2 by 2 matrices have two eigenvector directions and two eigenvalues. we will show that det(a − λi)=0. this section explains how to compute the x’s and λ’s. it can come early in the course. we only need the determinant ad − bc of a 2 by 2 matrix. example 1 uses to find the eigenvalues λ = 1 and λ = det(a−λi)=0 1. The eigenvalues of aare precisely the solutions of λ in det(a−λi) = 0. (3) the above equation is called the characteristic equation of a. lemma 1. an n×n matrix acan have at most n distinct eigenvalues. proof. the characteristic polynomial of a is a polynomial of degree n. hence, equation (3) can have at most n distinct roots of λ.
Comments are closed.