Mastering Bias And Variance In Machine Learning Models Ml Optimization

Mastering Bias And Variance In Machine Learning Models Ml • get the guide for ai and ml governance → ibm.biz governance guides• explore our bias monitoring technology → ibm.biz ibm watsonx governance. You have likely heard about bias and variance before. they are two fundamental terms in machine learning and often used to explain overfitting and underfitting. if you're working with machine learning methods, it's crucial to understand these concepts well so that you can make optimal decisions in your own projects. in this article, you'll learn everything you need to know about bias, variance.
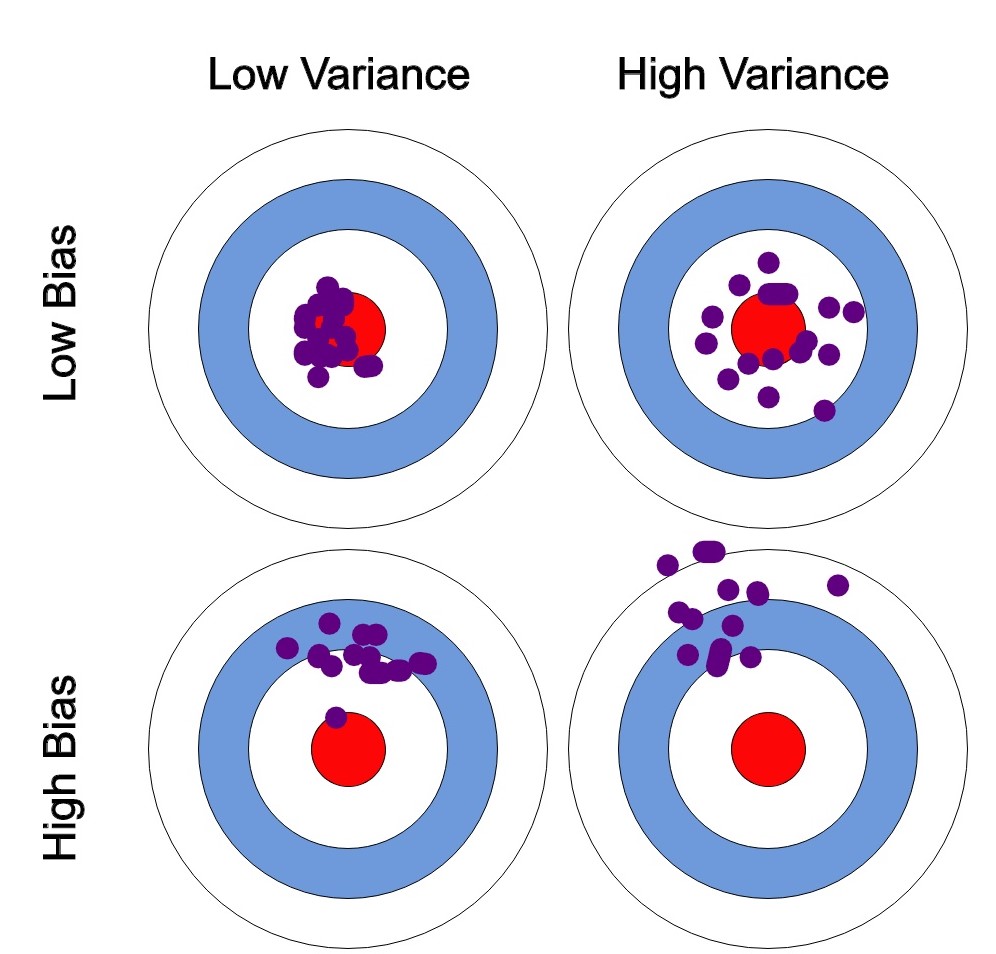
Bias Variance Machine Learning Master Supervised machine learning algorithms can best be understood through the lens of the bias variance trade off. in this post, you will discover the bias variance trade off and how to use it to better understand machine learning algorithms and get better performance on your data. let’s get started. update oct 2019: removed discussion of parametric nonparametric models (thanks alex). overview […]. Bias variance tradeoff is a design consideration when training the machine learning model. certain algorithms inherently have a high bias and low variance and vice versa. in this one, the concept of bias variance tradeoff is clearly explained so you make an informed decision when training your ml models. bias variance tradeoff – clearly. The bias variance tradeoff is a fundamental concept in machine learning and statistics that relates to the ability of a model to accurately capture the underlying patterns in a dataset. in essence, the bias variance tradeoff refers to the balance between the complexity of a model and its ability to generalize to new, unseen data. Bias. bias measures the discrepancy between the predictions made by our machine learning model and the true outcomes it aims to predict. this discrepancy arises because the model has learned from various datasets but is inherently restricted by its design and cannot perfectly mimic the true underlying pattern it tries to learn.

Bias Variance Trade Off In Machine Learning This Figure Illustrates The bias variance tradeoff is a fundamental concept in machine learning and statistics that relates to the ability of a model to accurately capture the underlying patterns in a dataset. in essence, the bias variance tradeoff refers to the balance between the complexity of a model and its ability to generalize to new, unseen data. Bias. bias measures the discrepancy between the predictions made by our machine learning model and the true outcomes it aims to predict. this discrepancy arises because the model has learned from various datasets but is inherently restricted by its design and cannot perfectly mimic the true underlying pattern it tries to learn. The performance of a machine learning model can be characterized in terms of the bias and the variance of the model. a model with high bias makes strong assumptions about the form of the unknown underlying function that maps inputs to outputs in the dataset, such as linear regression. a model with high variance is […]. A. the bias variance tradeoff in machine learning involves managing two types of errors. bias arises from overly simplistic models, leading to underfitting, while variance results from complex models capturing noise, causing overfitting. balancing these errors is crucial for creating models that generalize well to new data, optimizing.

Machine Learning Fundamentals Bias And Variance Youtube The performance of a machine learning model can be characterized in terms of the bias and the variance of the model. a model with high bias makes strong assumptions about the form of the unknown underlying function that maps inputs to outputs in the dataset, such as linear regression. a model with high variance is […]. A. the bias variance tradeoff in machine learning involves managing two types of errors. bias arises from overly simplistic models, leading to underfitting, while variance results from complex models capturing noise, causing overfitting. balancing these errors is crucial for creating models that generalize well to new data, optimizing.
Comments are closed.