Ml Observability Advanced Metrics Course Arize University
Ml Observability Advanced Metrics Course Arize University As more machine learning models are deployed into production, it’s imperative to have the right skillset to monitor, troubleshoot, and explain model performance. our self paced, advanced metrics certification course is designed help data scientists and ml practitioners gain confidence taking their models from research to production. through. Welcome to arize university’s ml observability advanced metrics certification course! this self guided course will teach you intuition, math, and best practices for effective machine learning observability across a variety of use cases. learn how to calculate the most common metrics in model monitoring – including performance, drift.
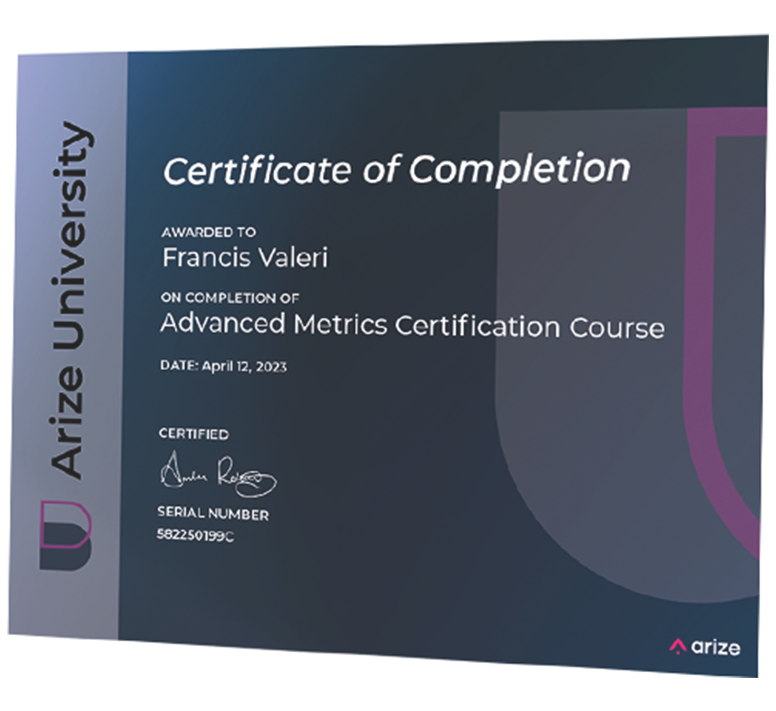
Ml Observability Advanced Metrics Course Arize Ai Ml observability advanced metrics intro lecture quiz lab unit 1: performance metrics in production congratulations on completing the course! next steps lecture. This course: includes math and python. can be taken in parts (each lesson is its own self contained primer) is best taken after completing or in tandem with our ml observability fundamentals certification course. here is a quick overview of the course: after a quick overview on observability, model evaluation metrics covers the intuition, math. Ml observability. ml observability fundamentals certification free ml observability advanced metrics course available until . amber roberts % complete free. Learn what constitutes model drift, how to monitor for drift in machine learning models, the types of drift — including concept drift, feature drift, and upstream drift — and drift resolution techniques. common terms in data science and machine learning demystified for ml practitioners. learn to build trustworthy machine learning models.
Comments are closed.