R Decision Trees The Best Tutorial On Tree Based Modeling In R

R Decision Trees The Best Tutorial On Tree Based Modeling In R The decision tree techniques can detect criteria for the division of individual items of a group into predetermined classes that are denoted by n. in the first step, the variable of the root node is taken. this variable should be selected based on its ability to separate the classes efficiently. The output diagram of the rpart.plot function shows a decision tree representation of the model. in this diagram, each node represents a split in the decision tree based on the predictor variables. the output diagram includes several pieces of information that can help us interpret the decision tree: the tree diagrams start with the node.
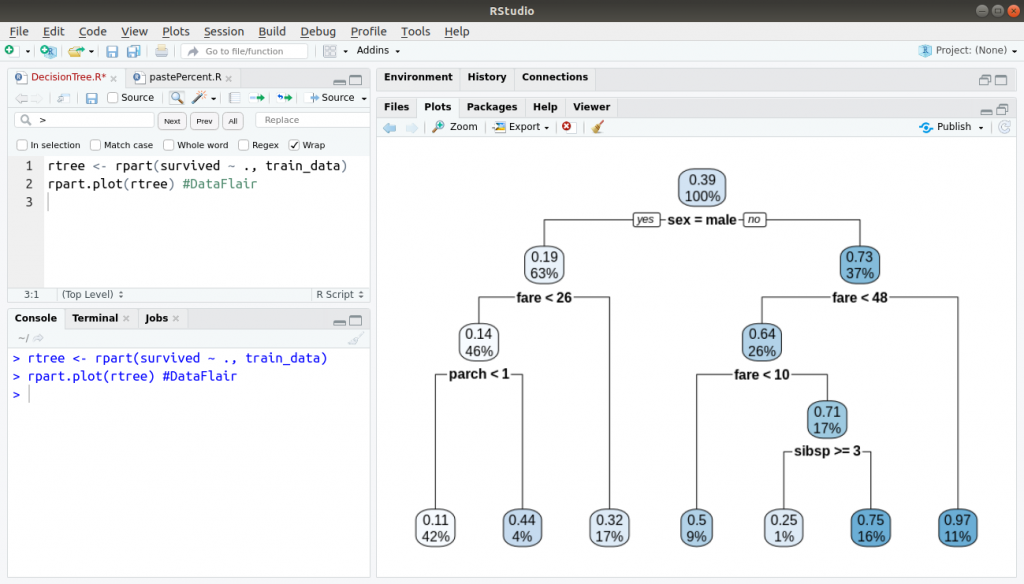
R Decision Trees The Best Tutorial On Tree Based Modeling In R Decision trees are also useful for examining feature importance, ergo, how much predictive power lies in each feature. you can use the varimp () function to find out. the following snippet calculates the importances and sorts them descendingly: the results are shown in the image below: image 5 – feature importances. Here's how to train the model: model < rpart ( species ~ ., data = train set, method = "class") model. the output of calling model is shown in the following image: image 3 decision tree classifier model from this image alone, you can see the "rules" decision tree model used to make classifications. Introduction to decision trees. decision trees are intuitive. all they do is ask questions, like is the gender male or is the value of a particular variable higher than some threshold. based on the answers, either more questions are asked, or the classification is made. simple! to predict class labels, the decision tree starts from the root. Training and visualizing a decision trees in r. to build your first decision tree in r example, we will proceed as follow in this decision tree tutorial: step 1: import the data. step 2: clean the dataset. step 3: create train test set. step 4: build the model. step 5: make prediction.
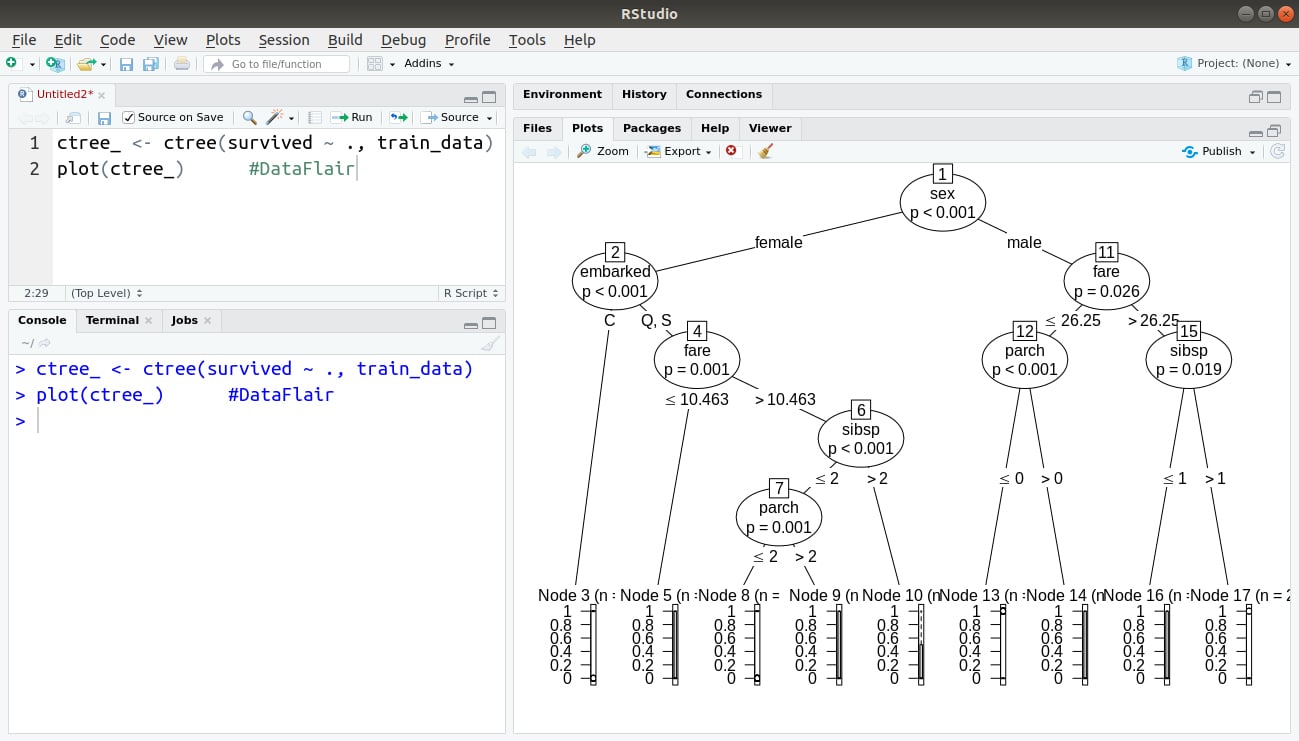
R Decision Trees The Best Tutorial On Tree Based Modeling In R Introduction to decision trees. decision trees are intuitive. all they do is ask questions, like is the gender male or is the value of a particular variable higher than some threshold. based on the answers, either more questions are asked, or the classification is made. simple! to predict class labels, the decision tree starts from the root. Training and visualizing a decision trees in r. to build your first decision tree in r example, we will proceed as follow in this decision tree tutorial: step 1: import the data. step 2: clean the dataset. step 3: create train test set. step 4: build the model. step 5: make prediction. Library (rpart) #for fitting decision trees library (rpart.plot) #for plotting decision trees step 2: build the initial classification tree. first, we’ll build a large initial classification tree. we can ensure that the tree is large by using a small value for cp, which stands for “complexity parameter.”. 11.1 introduction. classification trees are non parametric methods to recursively partition the data into more “pure” nodes, based on splitting rules. logistic regression vs decision trees. it is dependent on the type of problem you are solving. let’s look at some key factors which will help you to decide which algorithm to use:.
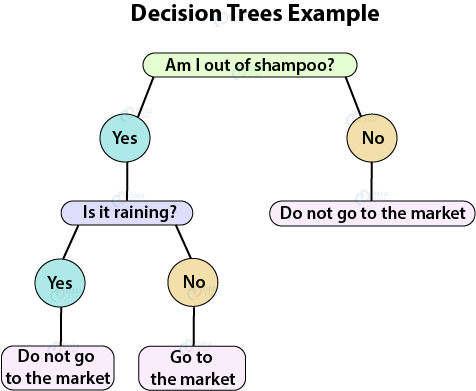
R Decision Trees The Best Tutorial On Tree Based Modeling In R Library (rpart) #for fitting decision trees library (rpart.plot) #for plotting decision trees step 2: build the initial classification tree. first, we’ll build a large initial classification tree. we can ensure that the tree is large by using a small value for cp, which stands for “complexity parameter.”. 11.1 introduction. classification trees are non parametric methods to recursively partition the data into more “pure” nodes, based on splitting rules. logistic regression vs decision trees. it is dependent on the type of problem you are solving. let’s look at some key factors which will help you to decide which algorithm to use:.
Comments are closed.