Regression Coefficient Formula Interpretation With Examples
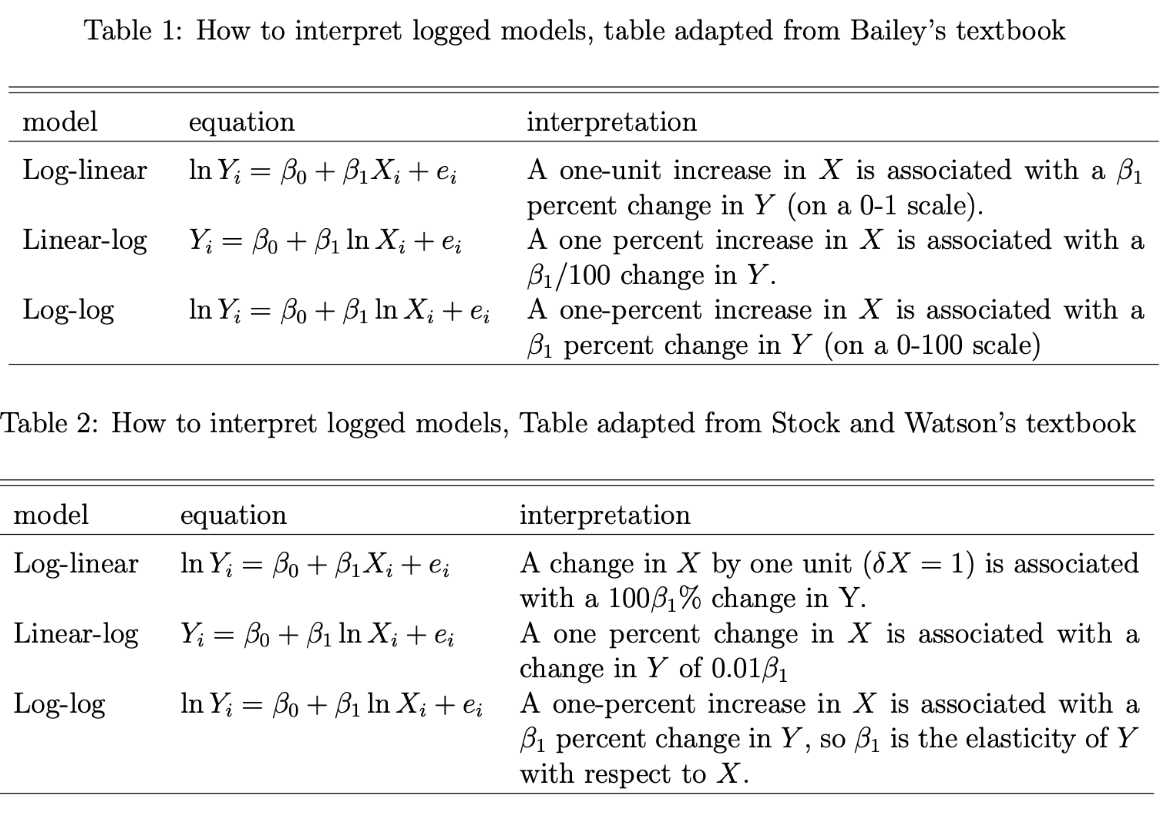
Interpretation Of Regression Coefficients With Examples Interpreting the intercept. the intercept term in a regression table tells us the average expected value for the response variable when all of the predictor variables are equal to zero. in this example, the regression coefficient for the intercept is equal to 48.56. this means that for a student who studied for zero hours (hours studied = 0. For example, suppose a simple regression equation is given by y = 7x 3, then 7 is the coefficient, x is the predictor and 3 is the constant term. suppose the equation of the best fitted line is given by y = ax b then, the regression coefficients formula is given as follows:.

Regression Coefficient Formula Interpretation With Examples A regression coefficient is the quantity that sits in front of an independent variable in your regression equation. it is a parameter estimate describing the relationship between one of the independent variables in your model and the dependent variable. in the simple linear regression below, the quantity 0.5, which sits in front of the variable. The height coefficient in the regression equation is 106.5. this coefficient represents the mean increase of weight in kilograms for every additional one meter in height. if your height increases by 1 meter, the average weight increases by 106.5 kilograms. the regression line on the graph visually displays the same information. A linear regression equation describes the relationship between the independent variables (ivs) and the dependent variable (dv). it can also predict new values of the dv for the iv values you specify. in this post, we’ll explore the various parts of the regression line equation and understand how to interpret it using an example. For example, if you have the linear regression equation y= 10 2x, the coefficient of 2 tells you that for every 1 unit increase in x, the model predicts that y will increase by 2. in a modified linear regression model or a nonlinear regression, the interpretation of the coefficients can start to change.
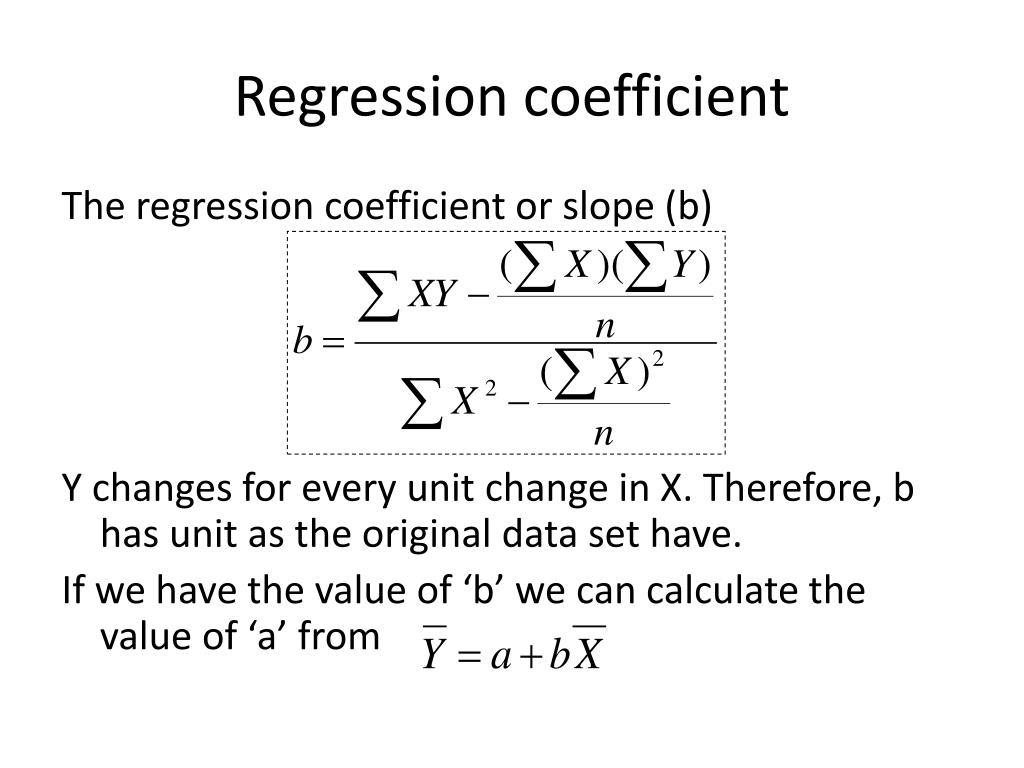
Ppt Simple Regression And Correlation Powerpoint Presentation Free A linear regression equation describes the relationship between the independent variables (ivs) and the dependent variable (dv). it can also predict new values of the dv for the iv values you specify. in this post, we’ll explore the various parts of the regression line equation and understand how to interpret it using an example. For example, if you have the linear regression equation y= 10 2x, the coefficient of 2 tells you that for every 1 unit increase in x, the model predicts that y will increase by 2. in a modified linear regression model or a nonlinear regression, the interpretation of the coefficients can start to change. This is the y intercept of the regression equation, with a value of 0.20. you can plug this into your regression equation if you want to predict happiness values across the range of income that you have observed: happiness = 0.20 0.71*income ± 0.018. the next row in the ‘coefficients’ table is income. The equation is estimated by converting the \(y\) values to logarithms and using ols techniques to estimate the coefficient of the \(x\) variable, \(b\). this is called a semi log estimation. again, differentiating both sides of the equation allows us to develop the interpretation of the \(x\) coefficient \(b\):.
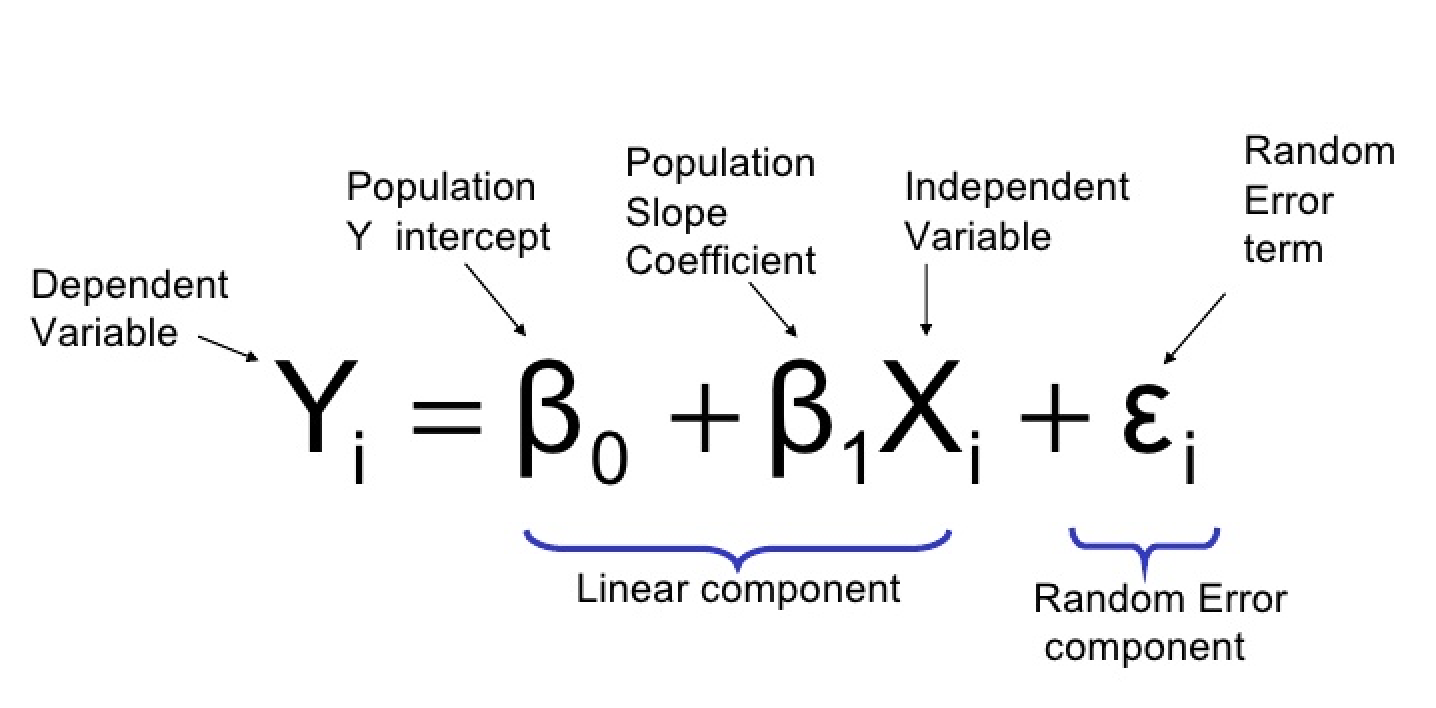
Regression Equation Sixsigma Dsi Lean Six Sigma Glossary Term This is the y intercept of the regression equation, with a value of 0.20. you can plug this into your regression equation if you want to predict happiness values across the range of income that you have observed: happiness = 0.20 0.71*income ± 0.018. the next row in the ‘coefficients’ table is income. The equation is estimated by converting the \(y\) values to logarithms and using ols techniques to estimate the coefficient of the \(x\) variable, \(b\). this is called a semi log estimation. again, differentiating both sides of the equation allows us to develop the interpretation of the \(x\) coefficient \(b\):.
Comments are closed.