Table 2 3 From Traffic And Road Sign Recognition Semantic Scholar
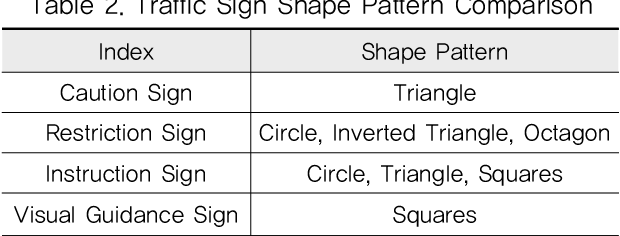
Table 2 From The Road Traffic Sign Recognition And Automatic This project is to implement a road sign recognition model based on ai and image analysis technologies, which applies a machine learning method, support vector machines, to recognize road signs. we focus on recognizing seven categories of road sign shapes and five categories of speed limit signs. two kinds of features, binary image and zernike. An overall comparative study of efficiency over the various machine learning and image processing methods used has been drawn, in spite of the much variety in the used database. in this survey, the need of traffic road safety has been discussed and an overview of traffic sign detection and recognition research works has been provided including novel, breakthrough approaches. traffic sign.
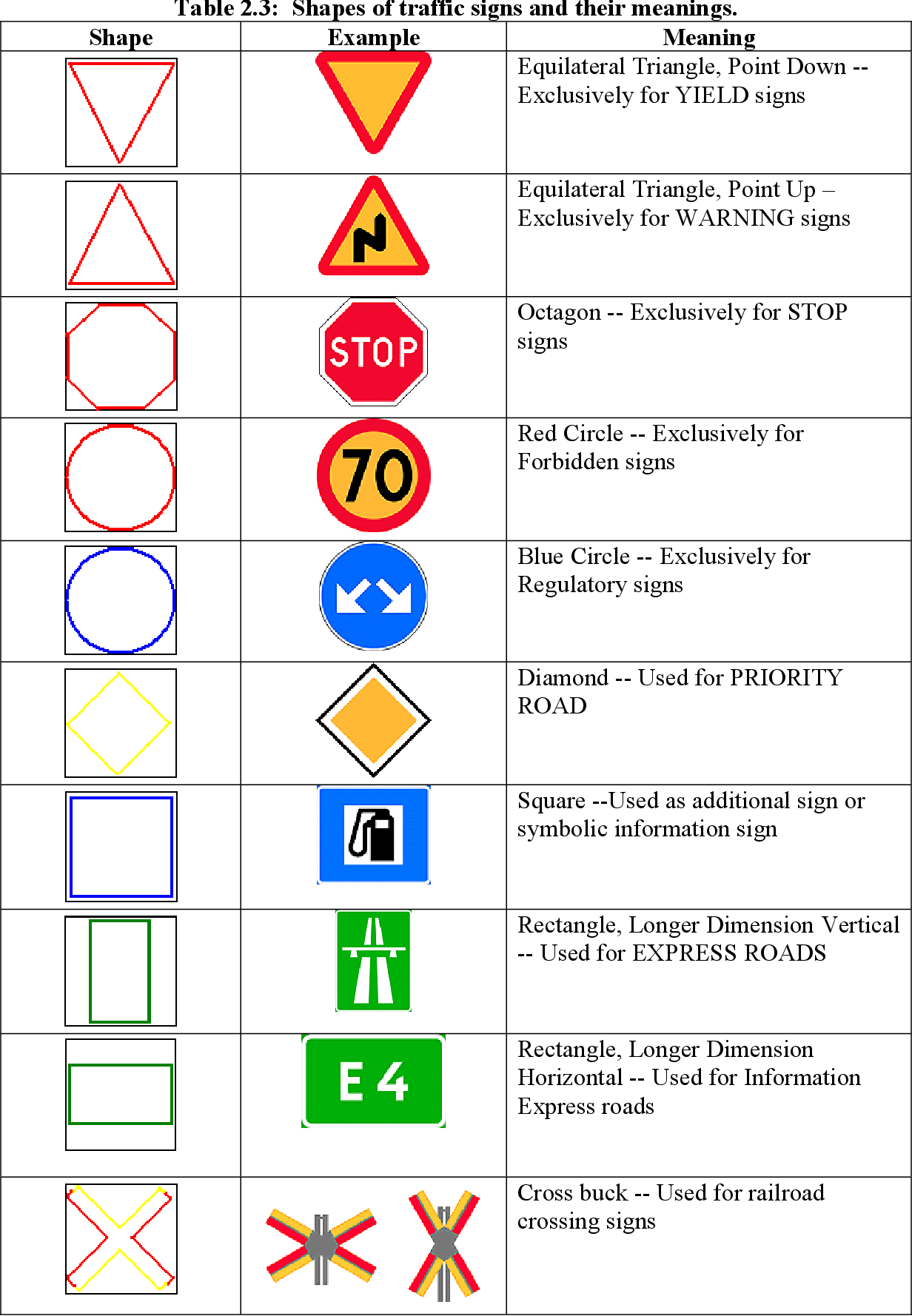
Table 2 3 From Traffic And Road Sign Recognition Semant Doi: 10.1109 icosp.2014.7015147 corpus id: 18469729; traffic sign recognition using hog svm and grid search @article{yao2014trafficsr, title={traffic sign recognition using hog svm and grid search}, author={chang yao and feng wu and hou jin chen and xiaoli hao and yan shen}, journal={2014 12th international conference on signal processing (icsp)}, year={2014}, pages={962 965}, url={ api. On the new test set of cctsdb 2021, for small objects, the precision is 88.1%, and the recall rate is 79.8%, compared with the traditional yolov5s model, it is improved by 12.5% and 23.9%. Traffic sign recognition is a complex and challenging yet popular problem that can assist drivers on the road and reduce traffic accidents. most existing methods for traffic sign recognition use convolutional neural networks (cnns) and can achieve high recognition accuracy. however, these methods first require a large number of carefully crafted traffic sign datasets for the training process. Traffic sign recognition (tsr) systems are essential for strengthening road safety, enhancing traffic management, and promoting efficient driving due to the ever increasing number of vehicles on the roads and the need for better transportation systems. modern intelligent transportation systems rely on tsr systems to help with the detection, categorization, and interpretation of traffic signs.
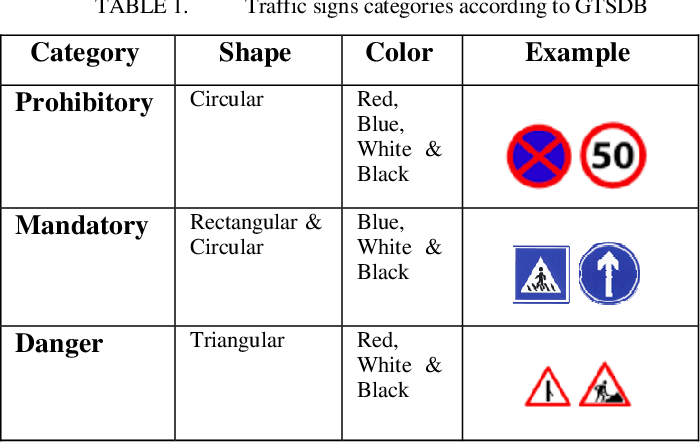
Table 1 From Traffic Signs Detection And Recognition System Using Deep Traffic sign recognition is a complex and challenging yet popular problem that can assist drivers on the road and reduce traffic accidents. most existing methods for traffic sign recognition use convolutional neural networks (cnns) and can achieve high recognition accuracy. however, these methods first require a large number of carefully crafted traffic sign datasets for the training process. Traffic sign recognition (tsr) systems are essential for strengthening road safety, enhancing traffic management, and promoting efficient driving due to the ever increasing number of vehicles on the roads and the need for better transportation systems. modern intelligent transportation systems rely on tsr systems to help with the detection, categorization, and interpretation of traffic signs. Traffic sign recognition (tsr) is one of the many utilities made possible by embedded systems with internet connections. through the usage of vehicular cameras, it’s possible to capture and classify traffic signs in real time with artificial intelligence (ai), more specifically, convolutional neural networks (cnns) based techniques. this article discusses the implementation of such tsr. The focal point of this research centers on the design and evaluation of a cnn based architecture trained on the german traffic sign recognition benchmark dataset, comprising over 50,000 annotated road sign images across 43 distinct classes. this research paper delves into the realm of road sign detection and classification using deep convolutional neural networks (cnns). leveraging the robust.
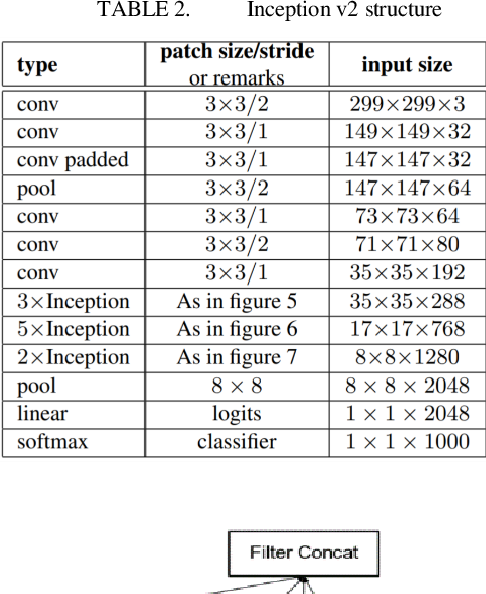
Pdf Traffic Signs Detection And Recognition System Using Deep Traffic sign recognition (tsr) is one of the many utilities made possible by embedded systems with internet connections. through the usage of vehicular cameras, it’s possible to capture and classify traffic signs in real time with artificial intelligence (ai), more specifically, convolutional neural networks (cnns) based techniques. this article discusses the implementation of such tsr. The focal point of this research centers on the design and evaluation of a cnn based architecture trained on the german traffic sign recognition benchmark dataset, comprising over 50,000 annotated road sign images across 43 distinct classes. this research paper delves into the realm of road sign detection and classification using deep convolutional neural networks (cnns). leveraging the robust.
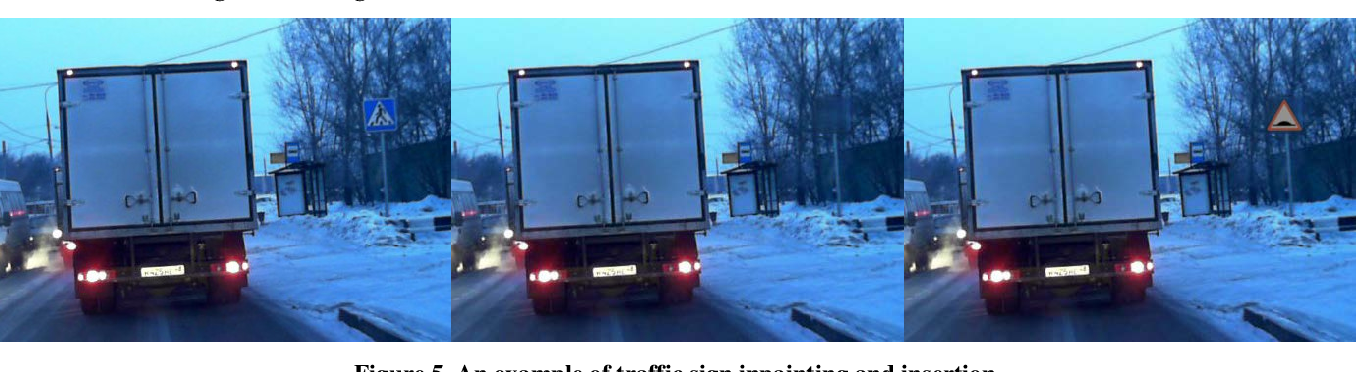
Figure 1 From Rare Traffic Sign Recognition Using Synthetic Training
Comments are closed.