The Effects Of Normalization Transformation On Loading Values For Pca
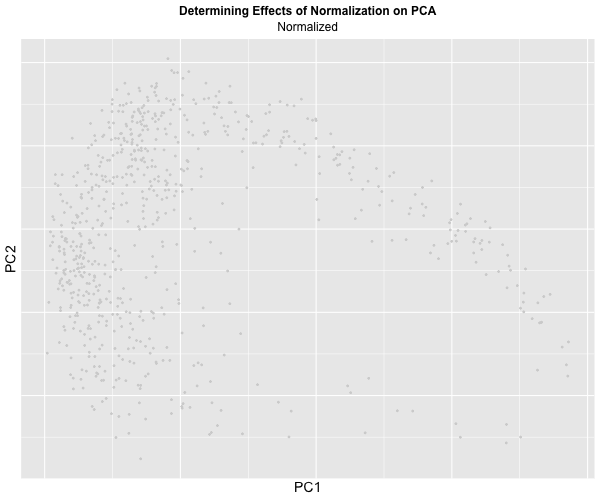
Animating The Effects Of Normalization Transformation On Loading For the bar plots graphing the loading values, the pc1 loading values for each specific gene is represented by the geometric primitive of lines and area. the categorical data of “gene name” is encoded by the visual channel of hue, with a distinct color distinguishing between the two genes that are also encoded for in the pca visualizations. Normalization is particularly useful when the data contains predefined boundaries, like image processing data. making the highest value 1 and the lowest value 0, or other selected values, all other values proportionally adjusted within this range. appropriateness for pca. pca assumes that the importance of a feature is determined by its variance.
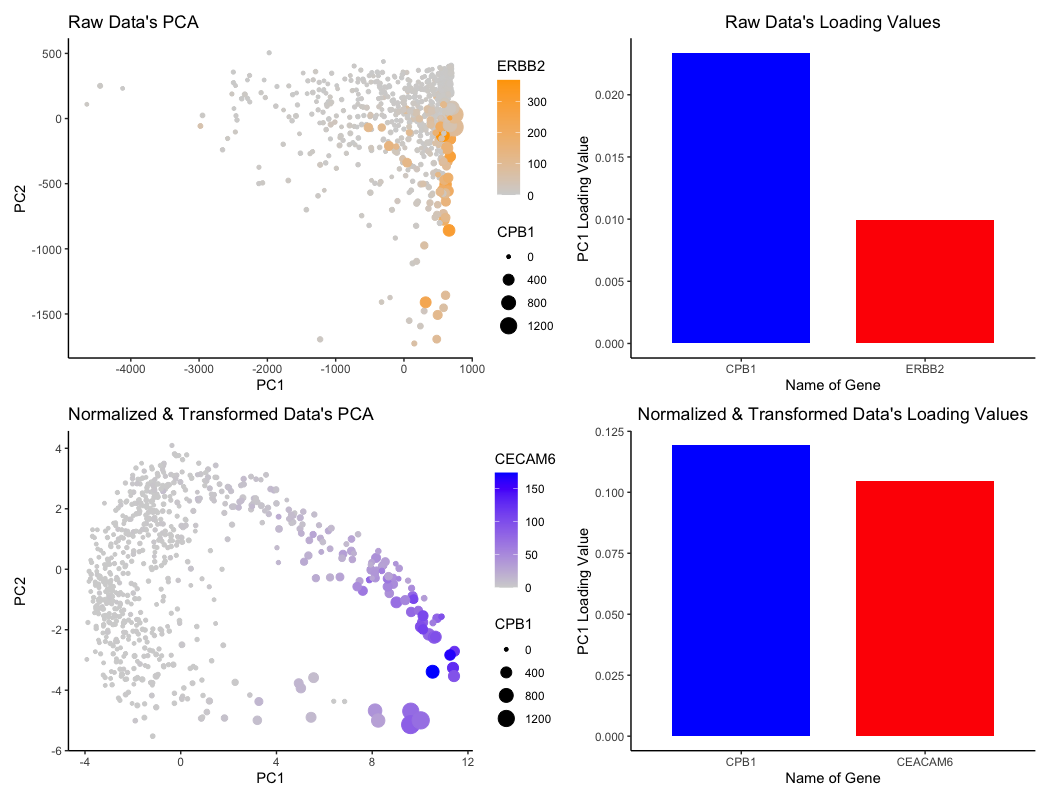
The Effects Of Normalization Transformation On Loading Values For Pca Figure description: i decided to animate the effects of normalization and transformation on pca by transitioning between two plots: (1) where the data is not normalized or transformed and (2) where the data is normalized and transformed. i had some difficulty with gganimate’s ability to actually display the pc1 and pc2 values on the x and y axis respectively and the ability to label the. The term normalization is used in many contexts, with distinct, but related, meanings. basically, normalizing means transforming so as to render normal. when data are seen as vectors, normalizing means transforming the vector so that it has unit norm. when data are though of as random variables, normalizing means transforming to normal. Note that the goal of my pca is to visualize the results of a clustering algorithm. fig 1 top 2 components with scaling. fig 2 top 2 components with normalizing. the correct term for the scaling you mean is z standardizing (or just "standardizing"). it is center then scale. Principal component analysis. the central idea of principal component analysis (pca) is to reduce the dimensionality of a data set consisting of a large number of interrelated variables, while retaining as much as possible of the variation present in the data set. this is achieved by transforming to a new set of variables, the principal.

Loading Plots Of Pca A Normalization With 1 Norm B Normalization Note that the goal of my pca is to visualize the results of a clustering algorithm. fig 1 top 2 components with scaling. fig 2 top 2 components with normalizing. the correct term for the scaling you mean is z standardizing (or just "standardizing"). it is center then scale. Principal component analysis. the central idea of principal component analysis (pca) is to reduce the dimensionality of a data set consisting of a large number of interrelated variables, while retaining as much as possible of the variation present in the data set. this is achieved by transforming to a new set of variables, the principal. Matrix representation: in the mathematical underpinnings of pca, loadings are connected with the eigenvectors of the covariance or correlation matrix of the data. these eigenvectors represent the loadings for the resultant principal components. when visualized in matrix form, each column corresponds to a specific principal component, and each. Importance of feature scaling. #. feature scaling through standardization, also called z score normalization, is an important preprocessing step for many machine learning algorithms. it involves rescaling each feature such that it has a standard deviation of 1 and a mean of 0. even if tree based models are (almost) not affected by scaling, many.

Loading Plots Of Pca A Normalization With 1 Norm B Normalization Matrix representation: in the mathematical underpinnings of pca, loadings are connected with the eigenvectors of the covariance or correlation matrix of the data. these eigenvectors represent the loadings for the resultant principal components. when visualized in matrix form, each column corresponds to a specific principal component, and each. Importance of feature scaling. #. feature scaling through standardization, also called z score normalization, is an important preprocessing step for many machine learning algorithms. it involves rescaling each feature such that it has a standard deviation of 1 and a mean of 0. even if tree based models are (almost) not affected by scaling, many.
Comments are closed.