Tinyml Continual Learning With Lwm2m

Tinyml Continual Learning With Lwm2m Continual learning refers to the ability of tinyml models to adapt over time. this can be accomplished by learning from new data sets without the need to retrain the model from scratch. although the techniques to realize continual learning are well known, practical implementations are often missing when running the models on resource. In the last few years, research and development on deep learning models and techniques for ultra low power devices in a word, tinyml has mainly focused on a train then deploy assumption, with static models that cannot be adapted to newly collected data without cloud based data collection and fine tuning. latent replay based continual learning (cl) techniques[1] enable online, serverless.
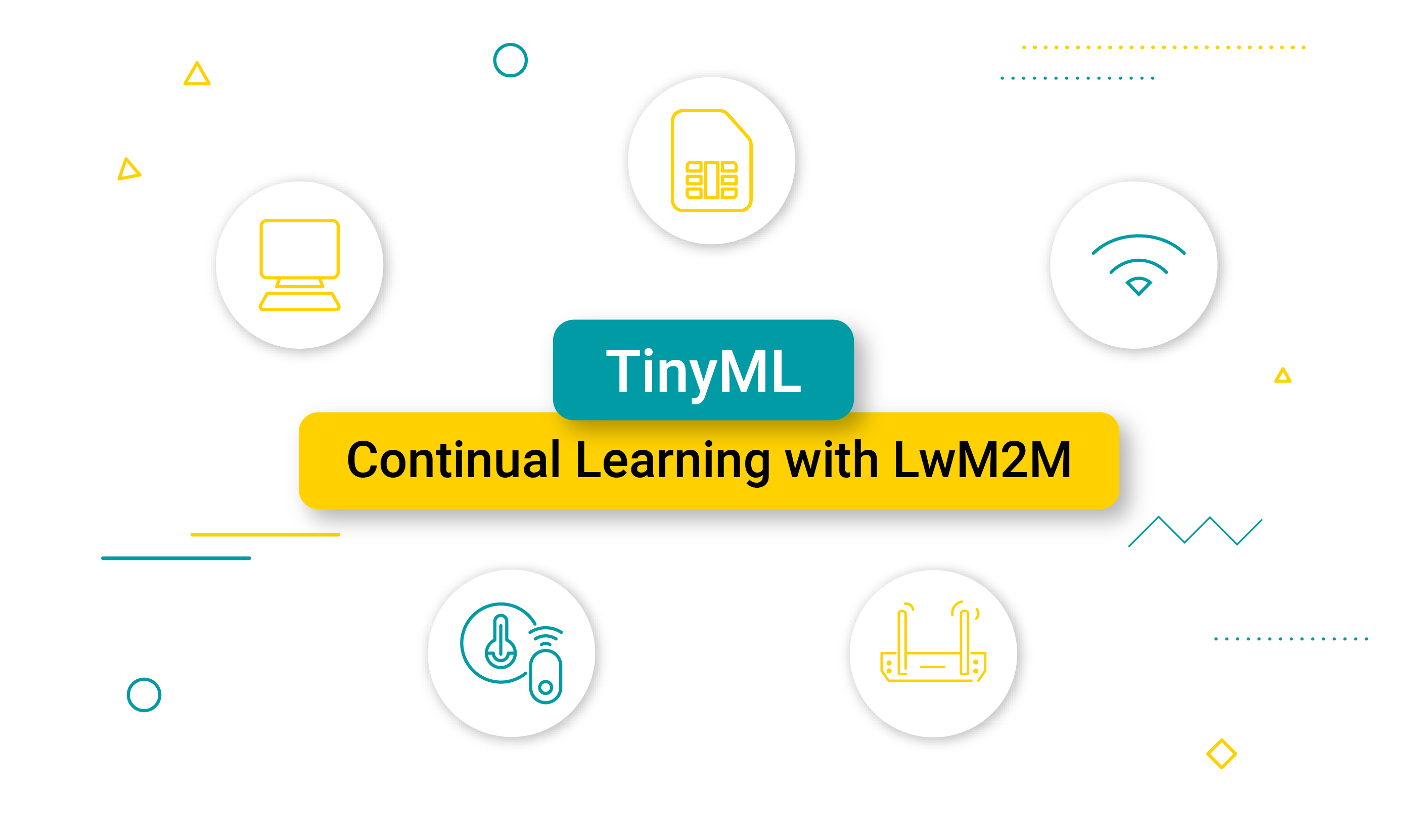
Tinyml Continual Learning With Lwm2m A tinyml platform for on device continual learning with quantized latent replays abstract: in the last few years, research and development on deep learning models & techniques for ultra low power devices– in a word, tinyml – has mainly focused on a train then deploy assumption, with static models that cannot be adapted to newly collected. In this work, we introduce a hw sw platform for end to end cl based on a 10 core fp32 enabled parallel ultra low power (pulp) processor. we rethink the baseline latent replay cl algorithm, leveraging quantization of the frozen stage of the model and latent replays (lrs) to reduce their memory cost with minimal impact on accuracy. When learning a new mini batch of data once every minute. index terms—tinyml, continual learning, deep neural networks, parallel ultra low power, microcontrollers. i. introduction the internet of things ecosystem is made possible by miniaturized and smart end node devices, which can sense the surrounding environment and take decisions based. Tiny machine learning projects.
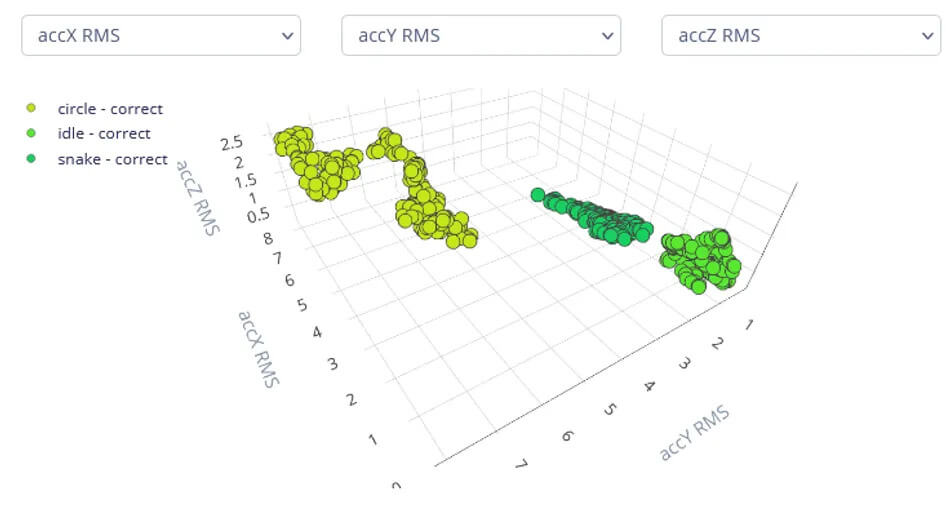
Tinyml Continual Learning With Lwm2m When learning a new mini batch of data once every minute. index terms—tinyml, continual learning, deep neural networks, parallel ultra low power, microcontrollers. i. introduction the internet of things ecosystem is made possible by miniaturized and smart end node devices, which can sense the surrounding environment and take decisions based. Tiny machine learning projects. The goal of on device learning (odl) is to make edge devices “smarter” and more efficient by observing changes in the data collected and self adjusting reconfiguring the device’s operating model. optionally the “knowledge” gained by the device is shared with other deployed devices. registration. august 31 september 1, 2022. Continual learning (cl) algorithms: •cl enables training also on tinyml platforms, with very bounded memory and computational resources. •continual learning with latent replays is our choice, since offers a solution compatible with embedded devices resources. “survey and benchmarking of machine learning accelerators”, reuther a. et al.
Comments are closed.