Weighted Least Squares Regression Calibration
Calibration Plot Showing Weighted Least Squares Regression Line When a least squares linear regression is used to fit experimental data to a linear calibration curve, equal emphasis is given to the variability of data points throughout the curve. however, because the absolute variation (as opposed to % error) is larger for higher concentrations, the data at the high end of the calibration curve tend to. Because the standard deviation for the signal, s std, is smaller for smaller concentrations of analyte, c std, a weighted linear regression gives more emphasis to these standards, allowing for a better estimate of the y intercept. figure 5.4.7 : a comparison of the unweighted and the weighted normal calibration curves.
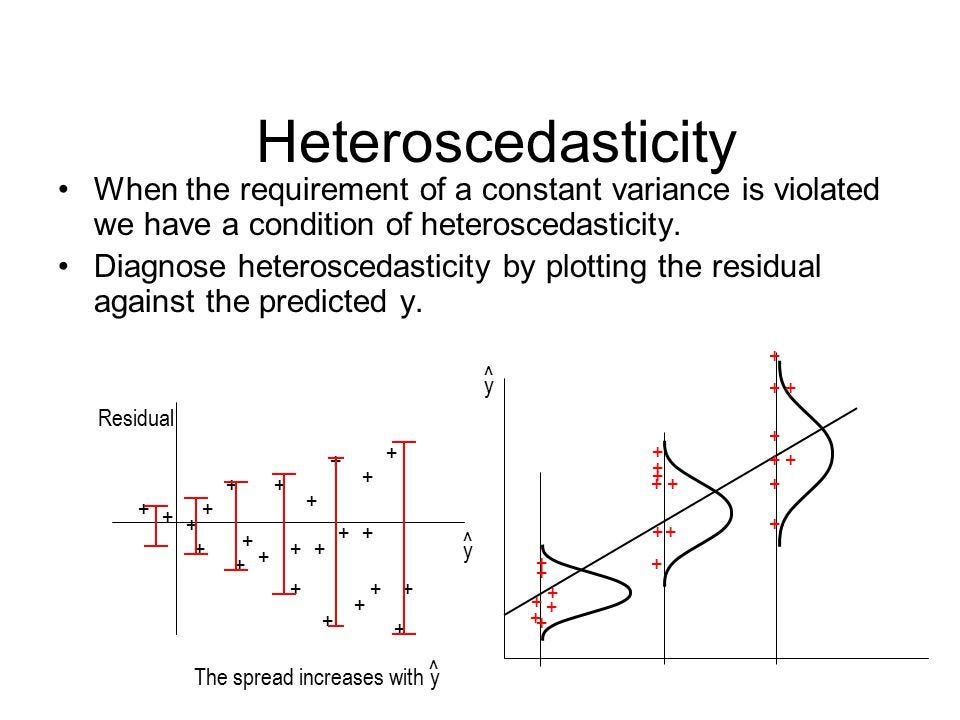
When And How To Use Weighted Least Squares Wls Models • the linear regression model is y i = β 0 β 1x i1 β px ip ε i, where the random errors are iid n(0,σ2). • what if the ε i’s are indep. w unequal var n(0,σ2 i)? • the ordinary least squares (ols) estimates for β j’s remain unbiased, but no longer have the minimum variance. • weighted least squares (wls) fixes the. Figure 1: unweighted linear regression model for the data in table 1. on the face of it, the regression co efficient (r2) seems to indicate linearity and the data seems to fit the regression model (trend line in figure 1). however, a simple ‘eyeball’ of the regression results does not allow us to properly investigate the validity of the model. In other words, we should use weighted least squares with weights equal to 1 s d 2. the resulting fitted equation from minitab for this model is: progeny = 0.12796 0.2048 parent. compare this with the fitted equation for the ordinary least squares model: progeny = 0.12703 0.2100 parent. A simple procedure for selecting the correct weighting factors for linear and quadratic calibration curves with least squares regression algorithm in bioanalytical lc ms ms assays is reported. the correct weighting factor is determined by the relationship between the standard deviation of instrument responses (σ) and the concentrations (x). the weighting factor of 1, 1 x, or 1 x2 should be.

Pdf Statistical Methods In Remote Sensing In other words, we should use weighted least squares with weights equal to 1 s d 2. the resulting fitted equation from minitab for this model is: progeny = 0.12796 0.2048 parent. compare this with the fitted equation for the ordinary least squares model: progeny = 0.12703 0.2100 parent. A simple procedure for selecting the correct weighting factors for linear and quadratic calibration curves with least squares regression algorithm in bioanalytical lc ms ms assays is reported. the correct weighting factor is determined by the relationship between the standard deviation of instrument responses (σ) and the concentrations (x). the weighting factor of 1, 1 x, or 1 x2 should be. Weighted least square regression. one of the common assumptions underlying most process modeling methods, including linear and nonlinear least squares regression, is that each data point provides equally precise information about the deterministic part of the total process variation. in other words, it is assumed that the standard deviation of. In order to counteract the greater influence of the greater concentrations on the fitted regression line, the weighted least squares linear regression is used. the expression to be minimised now takes the following form [1]: (2) ss =∑ (y observed,i −y predicted,i) 2 σ 2 i where σ i 2 is the variance of the standard point.
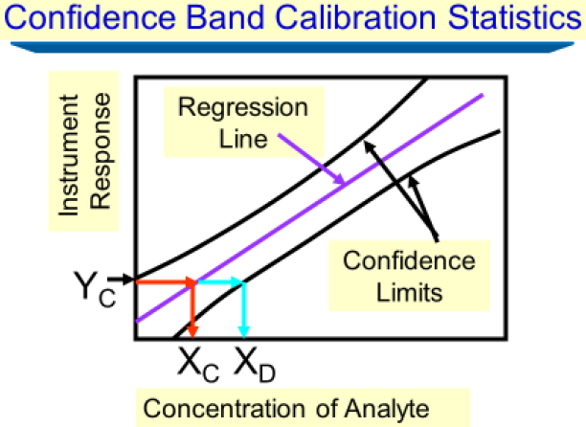
Use Of Weighted Least Squares And Confidence Band Calibration Weighted least square regression. one of the common assumptions underlying most process modeling methods, including linear and nonlinear least squares regression, is that each data point provides equally precise information about the deterministic part of the total process variation. in other words, it is assumed that the standard deviation of. In order to counteract the greater influence of the greater concentrations on the fitted regression line, the weighted least squares linear regression is used. the expression to be minimised now takes the following form [1]: (2) ss =∑ (y observed,i −y predicted,i) 2 σ 2 i where σ i 2 is the variance of the standard point.

Selecting The Correct Weighting Factors For Linear And Quadratic
Comments are closed.